LAS Publishes SCADS 2022 Technical Report
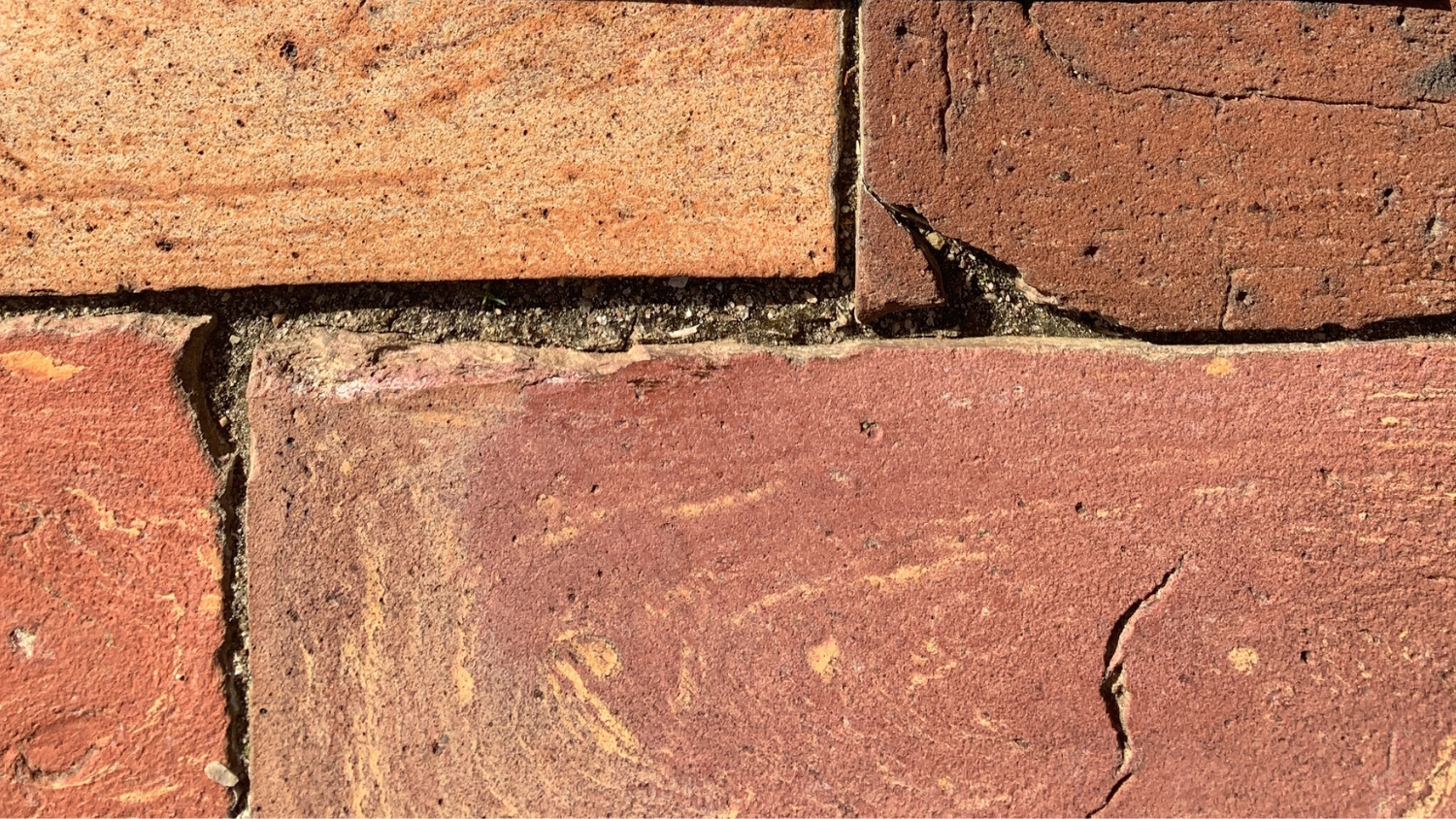
The foundational report details key takeaways from the lab’s inaugural 8-week summer conference.
Short, customized, instantaneous reports for intelligence analysts are feasible, but there’s still more work to be done, according to a new report on the research findings from the 2022 Summer Conference on Applied Data Science. The conference, also called SCADS, was the first of its kind for the Laboratory for Analytic Sciences at NC State.
The conference brought 40 industry, academic and government professionals together in Raleigh, N.C., to attack a mission-relevant grand challenge: how do we use artificial intelligence and machine learning to generate tailored daily reports (TLDRs) for individuals that capture information relevant to their objectives and interests?
The 47-page SCADS technical report describes the work completed by the 2022 conference participants. It explains the progress made toward building a TLDR, then outlines next steps that could be done to continue, presumably by future SCADS participants over the next 5 to 10 years.
“One of the difficulties we face in creating a TLDR is that there is a huge amount of information available in a variety of formats, and we need to identify which portions of that information are relevant, or not, to each knowledge worker,” says Liz Richerson, senior research scholar at LAS and lead author of the report. “Part of SCADS research has been looking at how we can teach a machine – based on a user’s behavior – to quickly find information that may be of interest to them in an operational environment.”
Here, the operational environment means a national security-related setting or situation, but the concept of the TLDR is broadly applicable and could be used by lawyers, healthcare professionals, or anyone who works with large quantities of digital data.
Technical takeaways from the report include:
- Graph-to-text case study: To best tell the story of the information contained in a knowledge graph, SCADS participants developed a proof of concept that takes a knowledge graph and returns a tailored text summary, presenting only the information that is of interest to the user. Examples of a knowledge graph could include social networks or a supply chain. Knowledge graphs make it easy to visualize relationships. This approach could be applied to technical networks too, like visualizing which bank accounts are transferring funds to understand where money is going.
- Recommender system proof of concept: SCADS participants proposed a best-performing news recommendation algorithm that could be used for the TLDR. They outlined issues for users to be aware of, like keeping a broad view so that the system continues to incorporate new content, not just the types of content on which a user has already clicked. Participants also included an explanation of why certain things were recommended. For example, a list of words in a title that are similar to other things that a user has looked at show why the system recommended it.
Richerson says participants assessed various algorithms and models to determine their usefulness for a TLDR.
“In some cases, this involved creating prototype systems that mimicked eventual TLDR functionality,” she says. “In other cases, researchers determined something was not useful for a TLDR and, often, came up with new research questions to investigate in the future. While we did not solve the grand challenge during the inaugural conference, we did make foundational progress on problems associated with the challenge and creating a TLDR.”
In June, a new group of SCADS researchers will arrive on campus to continue building upon the work.
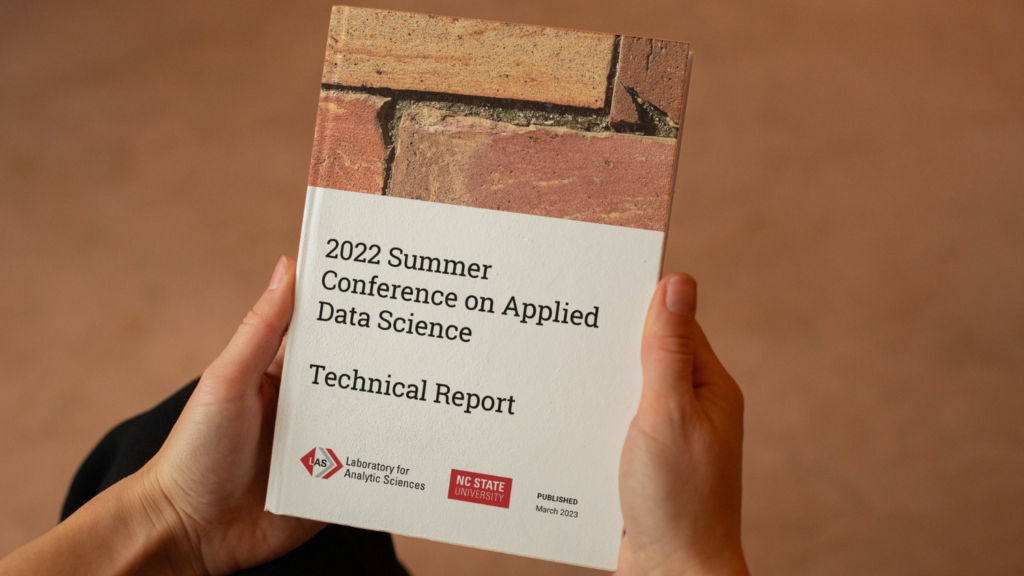
- Categories: