Laboratory for Analytic Sciences Publishes SCADS 2023 Technical Takeaways
Participants in the lab’s Summer Conference on Applied Data Science built AI-enabled prototypes for intelligence analysts, according to an executive summary of the results.
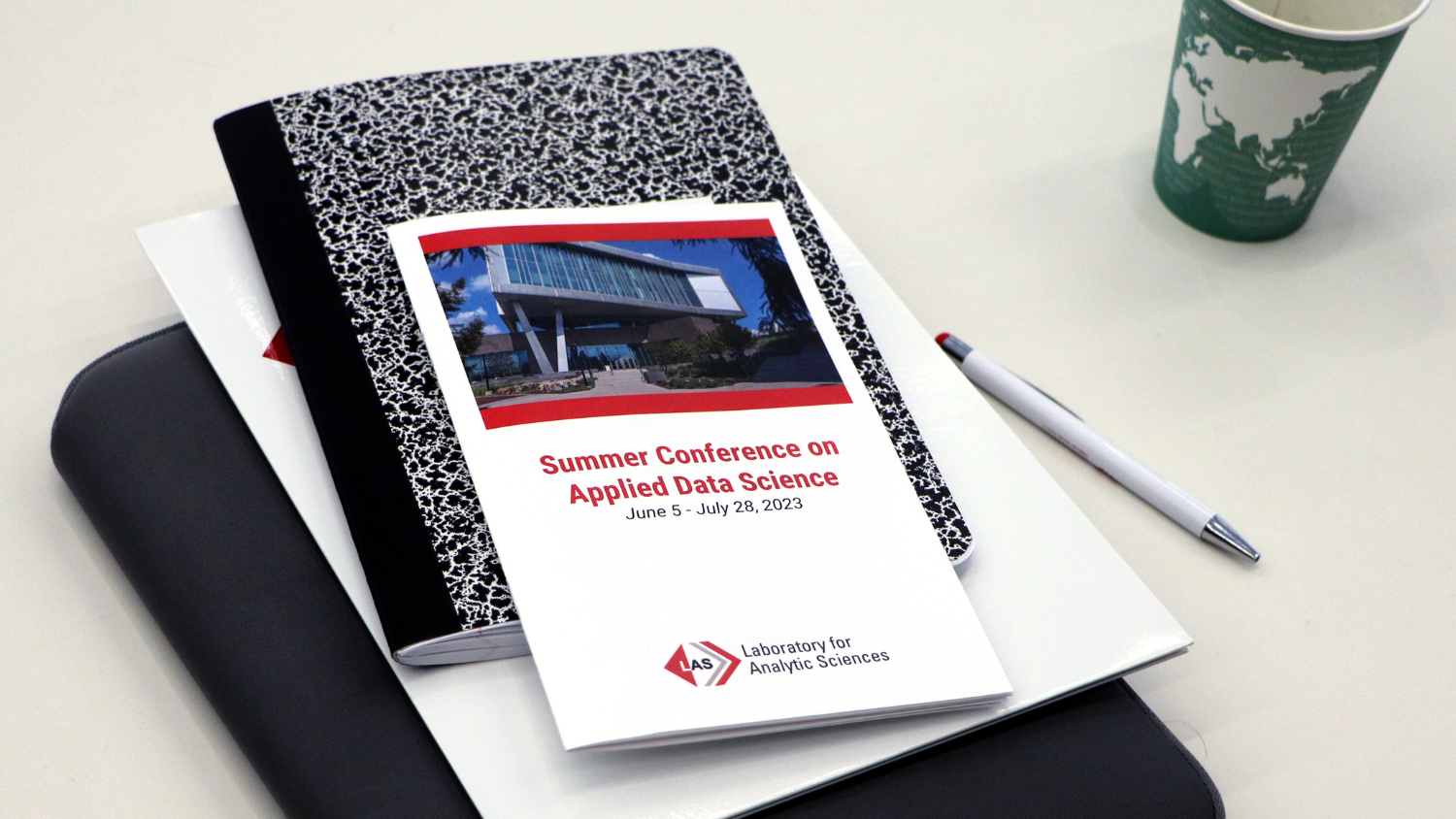
Data scientists created three preliminary prototypes that can be built upon and tested by next year’s participants, according to an executive summary of the findings from the second annual Summer Conference on Applied Data Science. The conference, also called SCADS, is organized by the Laboratory for Analytic Sciences at NC State University. Funding for the conference is provided through NC State’s partnership with the National Security Agency.
The conference brought 50 industry, academic, and government professionals together in Raleigh, N.C., to tackle a mission-relevant grand challenge: How do we generate tailored daily reports (TLDRs) for individuals that capture information relevant to their objectives and interests?
“Analysts are inundated with information,” says Liz Richerson, senior research scholar at LAS and lead author of the executive summary. “A tailored daily report will help people sift through data to find what’s relevant in order to make critical decisions.”
The National Security Commission on Artificial Intelligence (NSCAI) and the Center for Strategic and International Studies (CSIS) helped to inspire the grand challenge through their message of the need to transform national intelligence by adopting AI-enabled capabilities.
Five Technical Takeaways
The SCADS executive summary describes the work completed by the 2023 conference participants, including progress made toward building a TLDR, the top takeaways, and steps for future SCADS participants to consider in the next 3 to 8 years.
Technical takeaways from the SCADS 2023 summary include:
- SCADS researchers can use prototype TLDR systems to discover the path forward. Participants created three preliminary models of TLDRs that can be built upon and tested by future SCADS researchers.
- We developed a framework to understand how a TLDR system would meet analysts’ needs. This framework outlines the necessary characteristics of a hypothetical TLDR system, like
- efficiency,
- functionality,
- usability,
- trustworthiness, and
- relevance.
Developing metrics that can measure how well a TLDR demonstrates these characteristics should be a top priority for future SCADS participants.
- Incorporating large language models (LLMs) in the creation of TLDRs helps with summarization, but not recommendations. Next year’s SCADS researchers should carefully consider the task to which an LLM will be applied. Tasks should be clearly defined in a way that imposes constraints or guardrails on LLM behavior.
- The existing TLDR prototypes need to be larger in scale. To meet the needs of analysts, an effective TLDR system should be able to scale up the types and sources of information being used in the system, the number of users of the system, and the wide variety of information needs of those users. Researchers should also consider how increasing scale will affect a system’s performance, effectiveness, and customizability.
- TLDR user feedback is essential to further development. Researchers should continuously talk to analysts and other potential users of a TLDR system to see how it can be refined to best meet their needs.
“The incremental progress we’re making around components within the TLDR is viable now, even if we don’t have a fully operational TLDR,” says Richerson. “The lessons we’re learning about how people work and what they want the TLDR to do, and how well it works – we can apply a lot of that knowledge today.”
Wanted: Data Science Researchers
LAS is currently recruiting participants for next year’s Summer Conference on Applied Data Science. Researchers interested in working on a data science problem for the summer in Raleigh, NC, are invited to apply for SCADS 2024. Participants will have access to documentation and resources produced during previous SCADS.
> Related: TLDR: Like the President’s Daily Brief, But for Everyone
- Categories: