Intelligence Community Partners Peruse Prototypes at LAS Research Symposium
National security professionals gathered to demo the newest initiatives in machine learning, data triage and human-machine teaming.
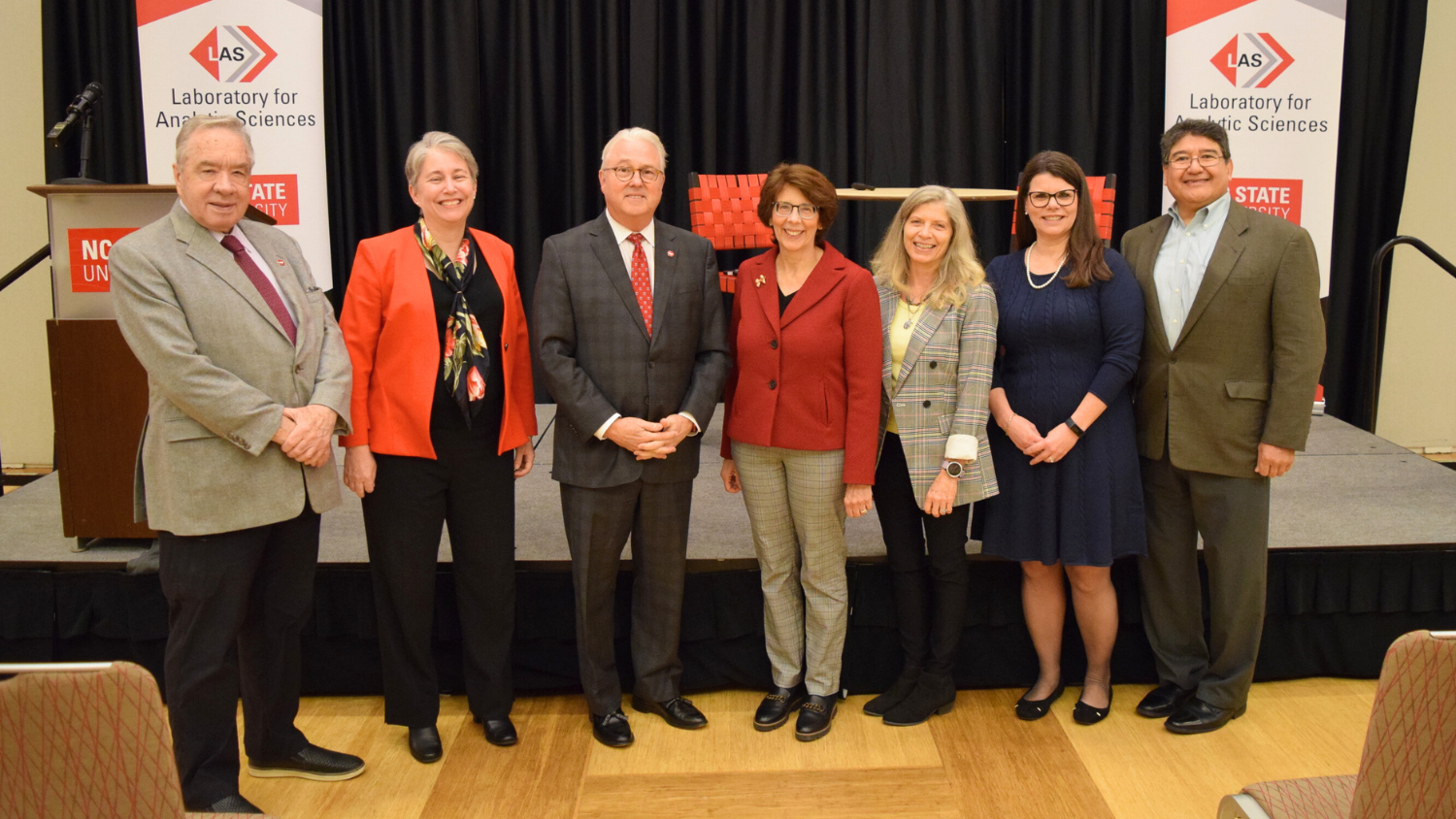
Imagine you’re watching video footage taken from the dashboard camera of a moving car. The video is grainy and the lighting isn’t ideal. The driver is going the speed limit, but the street scenes are passing by quickly.
It’s easy to spot larger objects in the video, like pedestrians and other cars. But you’re on a mission to find something smaller: security cameras in public places.
You’ve spotted a few cameras mounted to the corners of office buildings and residential front porches. After a while, though, your eyes need a break. You decide to hand off this arduous search task to your computer and say, “Hey A.I., please search this video for objects that look like security cameras while I go to lunch.” When you return to your desk, you eagerly take a look at the results. Some of the results are small objects that could be mistaken for cameras at first glance – things like street lamps, fans on air conditioning units, and windows.
After some fine-tuning to teach the computer exactly what you want, you run another search that returns better results: these are the security cameras you’re looking for.
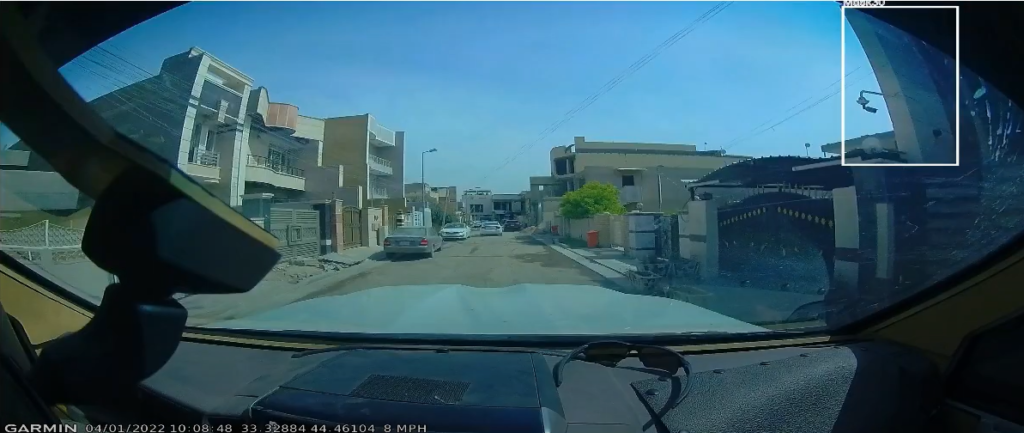
Last week, intelligence community partners from the U.S. Department of Defense gathered at NC State University to preview the newest initiatives in machine learning, AI, data triage and human-machine teaming developed by the Laboratory for Analytic Sciences (LAS). LAS academic, industry and government collaborators shared more than 30 demos and prototypes at the lab’s year-end research symposium. This year’s projects focused on using data to improve intelligence analysis through three major themes:
- Human-Machine Teaming: How can technology help analysts lighten their cognitive load?
- Operationalizing Machine Learning and Artificial Intelligence: How can advances like artificial intelligence be integrated into analysts’ workflows at scale?
- Content Triage: How can technology help analysts sort through huge amounts of data to find what is relevant for their analyses?
Catherine Aucella, executive director of the National Security Agency, spoke to more than 200 attendees at Talley Student Union and shared success stories from the NSA’s 10-year partnership with NC State.
“[The lab’s 8-week conference] SCADS led NSA to hold the first AI Augmented Sensemaking Leadership Summit hosted by LAS to build an integrated road map to meet NSA’s new strategy objectives,” Aucella said. She highlighted LAS’s engagement with students from minority-serving institutions in North Carolina and NC State’s computer science department, which allows NSA to recruit and retain a diverse workforce.
Jeannie Johnson, director of the Center for Anticipatory Intelligence at Utah State University, also spoke to attendees about how foresight and probability can be applied to combat security threats in the intelligence community.
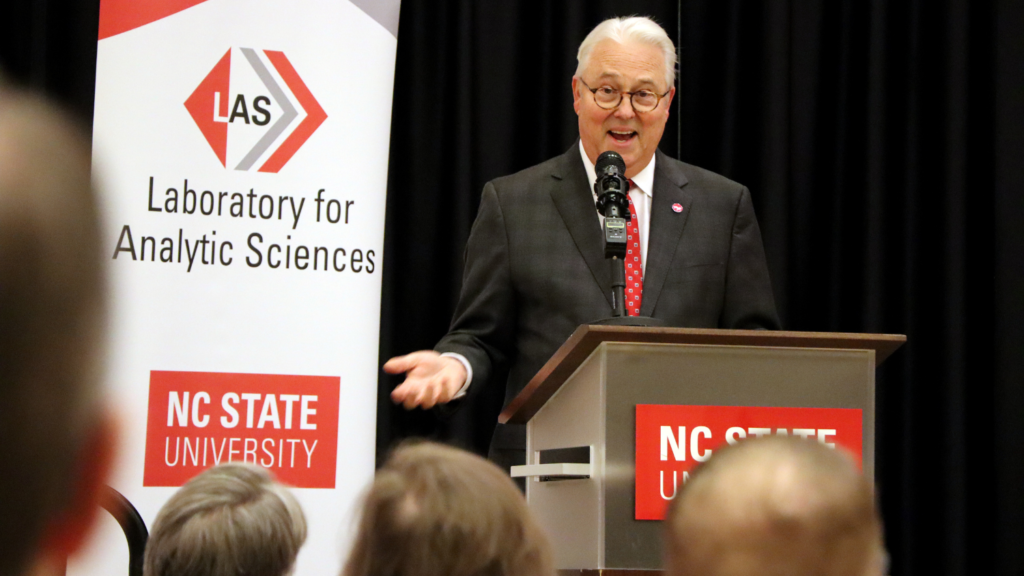
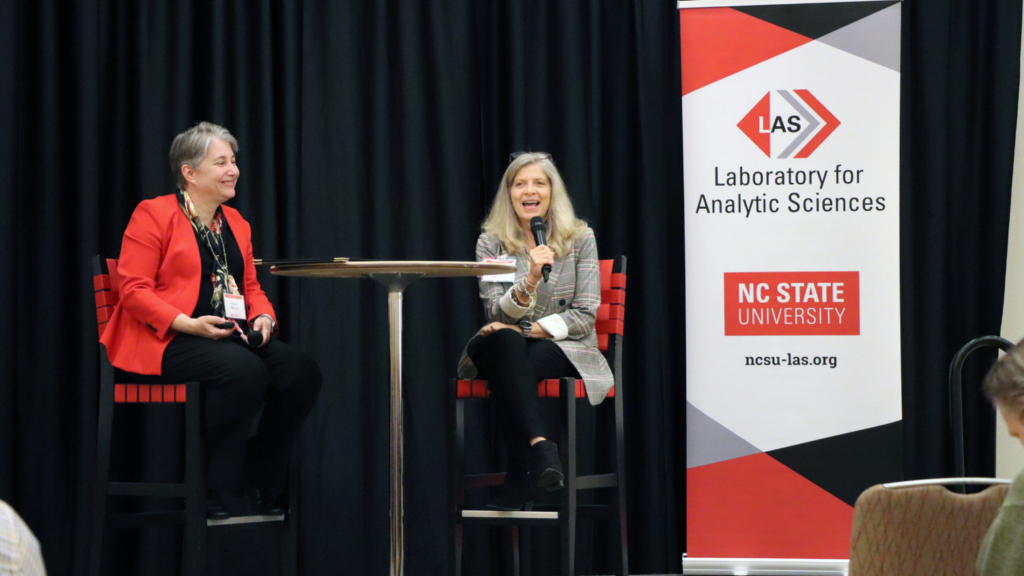
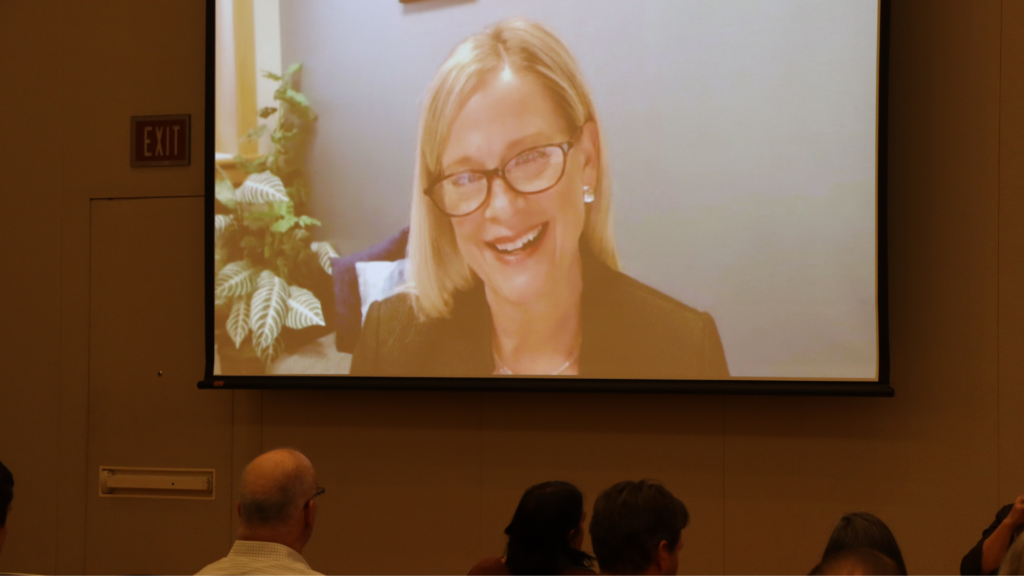
Putting Together a Puzzle
Human-Machine Teaming
The best technology saves time and removes tasks from your to-do list. Technological innovations have the potential to reduce the cognitive burden associated with processing and managing vast datasets. Their successful implementation hinges on a deep understanding of how humans can process the outputs and seamlessly integrate these technologies into their workflows. Projects in the human-machine teaming theme seek to understand how human analysts are partnering with emerging automated technologies to enhance their efficiency.
In the symposium’s first session, LAS hosted a panel discussion with Rob Capra, a professor at UNC School of Library and Information Science, Cara Widmer, a research scientist at Kairos Research, and Cayley Cruickshank DeFontes, a data scientist with the U.S. government.
“I like to think about human-machine teaming like I’m making dinner and I have a perfectly balanced, well-sharpened knife that … allows me to be the chef that I want to be,” Capra said. “A successful human-machine teaming to me is one where the human comes away saying, ‘this is a great tool.’”
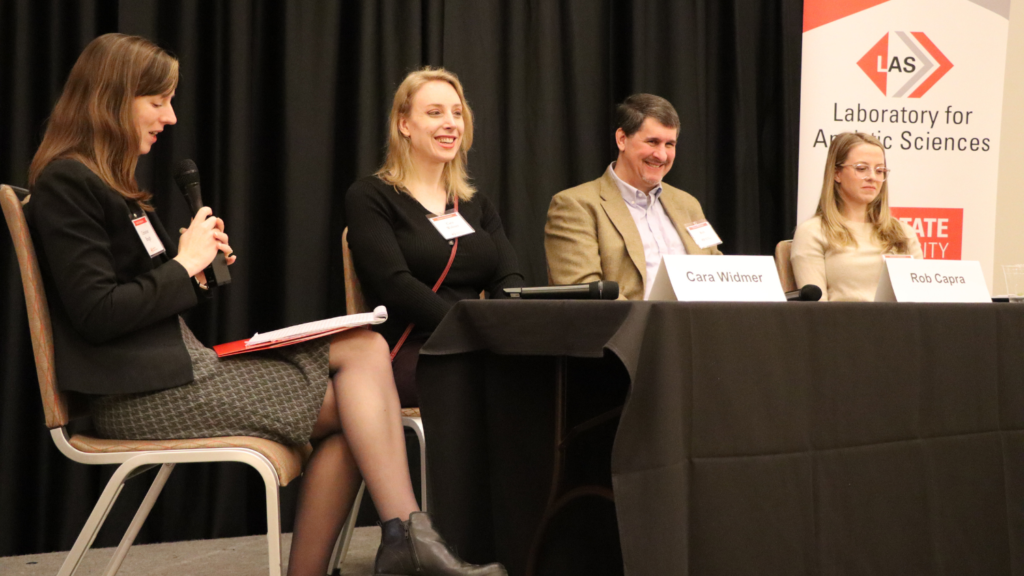
Forging Through Constraints
Operationalizing Artificial Intelligence and Machine Learning (AI/ML)
LAS research in this area focuses on how machine learning concepts and techniques can still be useful even when working under the constraints of an operational environment, like a person’s eye fatigue in the above example about dash cam video. Researchers examine the impact of those constraints on AI/ML performance when applied in operational settings. They also look for ways to reduce the costs of those constraints, whether they be financial, time, or cognitive resources. These efforts include creating data from which models can learn, training models, running models in realistic conditions, and sharing models for widespread use.
One of this year’s industry collaborators, SAS Institute, demonstrated the results of their research on training AI to search for the appearance of small CCTV security cameras in video footage. Watch their video about Object Detection Methods to Reduce Labeling Burden.
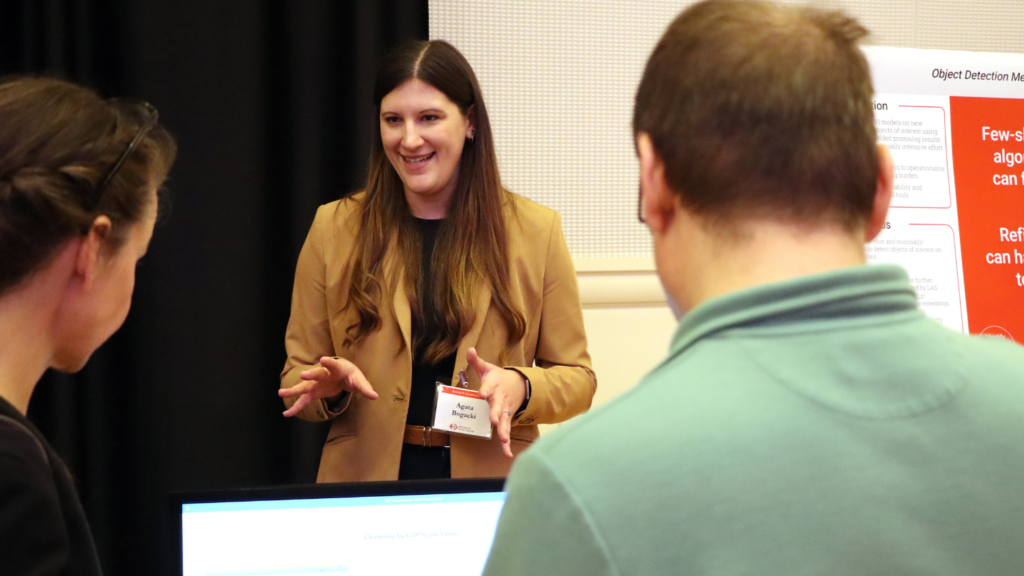
Sight, Sound, Search
Content Triage
Content triage research examines new, efficient methods to access and exploit large amounts of data, images, audio, and text. This includes semantic queries that help an intelligence analyst better navigate unknown information, improved use of knowledge graph structures, better ways to characterize voice, and flexible detection of sounds and images – all to enable analysts to retrieve unknown insights from data. After all, useful information that systems contain, but are never aware of, is perhaps the most tragic missed opportunity for the intelligence community’s prodigious efforts.
For example, students and faculty from Rochester Institute of Technology collaborated with LAS on a tool that would better help analysts discover fabricated videos, or deepfakes. Their deepfake detection tool project explores how to equip intelligence analysts with tools that match evolving digital manipulation technology, then align them with their unique needs and expertise.
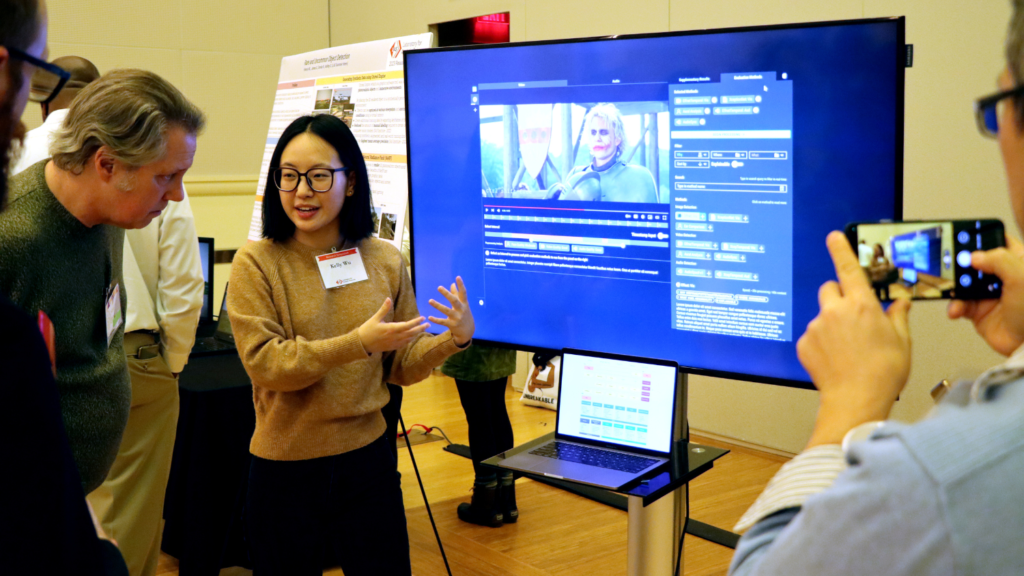
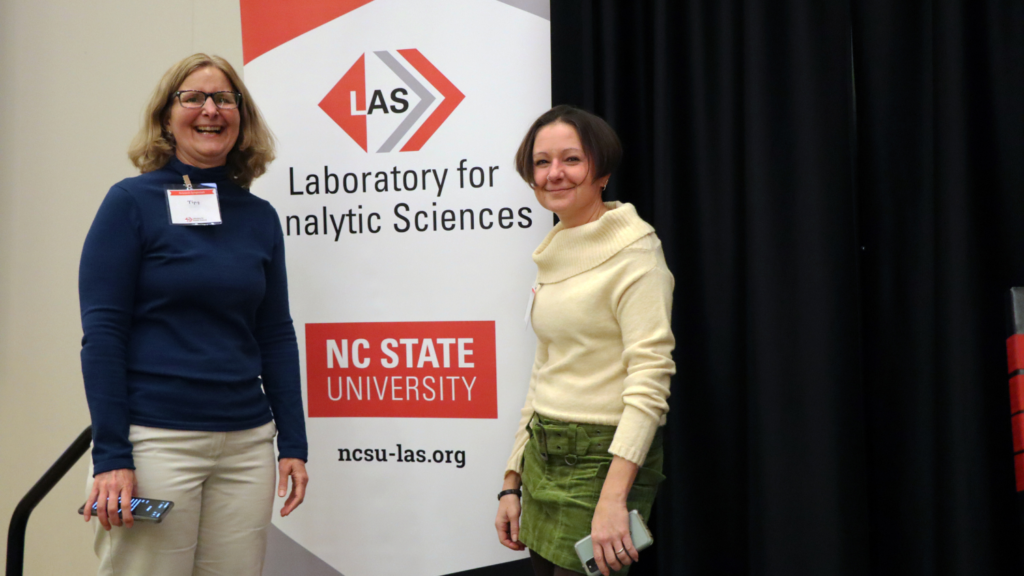
The Future of AI Research
Many of the projects on display encompass all three themes of content triage, operationalizing AI/ML, and human-machine teaming. One such project, GUESS (Gathering Unstructured Evidence with Semantic Search), explores how artificial intelligence and machine learning can help an analyst identify relevant information in poor speech-to-text transcriptions. When analysts are faced with a large volume of text to parse through, they can collaborate with GUESS to locate what’s needed. This prototype tool’s interactive workflow promotes alignment and trust calibration, demonstrating that the analyst’s ability to understand, interpret, and apply knowledge generated by AI/ML can help users find relevant information faster.
NSA’s executive director Aucella explained the importance of partnerships and collaborations like LAS in developing AI safely and securely.
“NSA cannot do this in a vacuum,” she said. “We need the assistance, perspectives and ideas of academia and industry.”
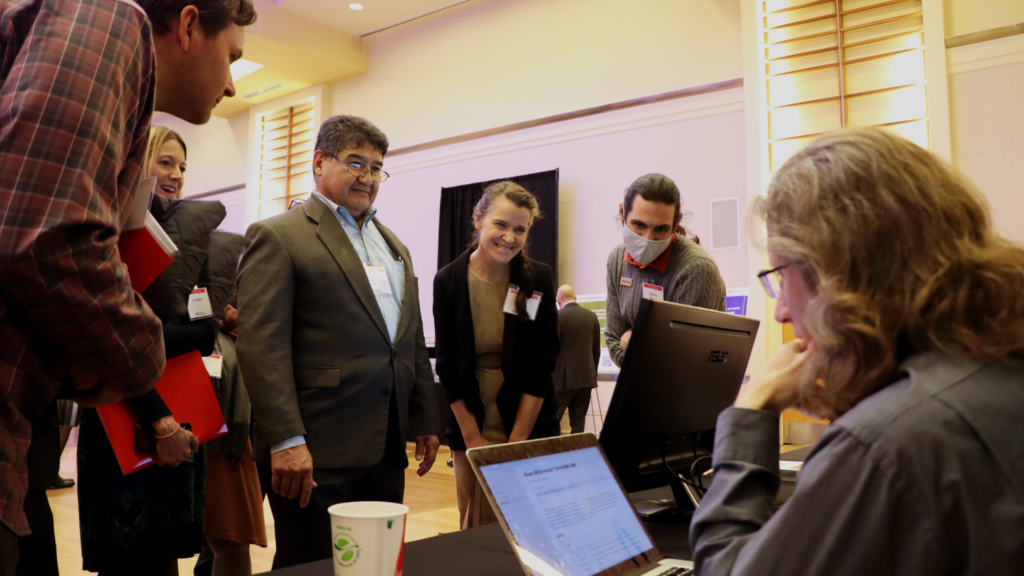
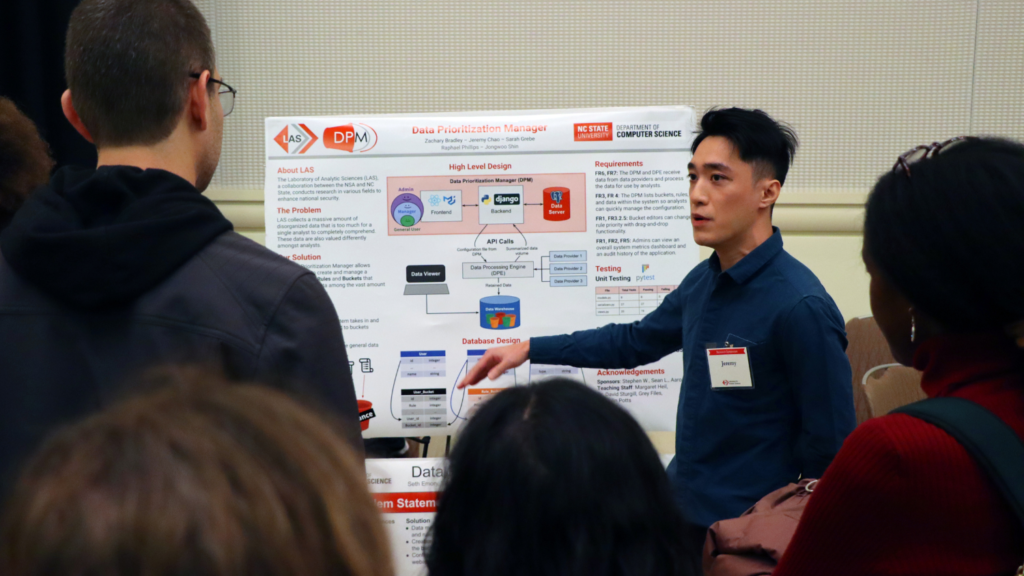
About LAS
The Laboratory for Analytic Sciences is a partnership between the intelligence community and North Carolina State University that develops innovative technology and tradecraft to help solve mission-relevant problems.
Founded in 2013 by the National Security Agency and NC State, each year LAS brings together collaborators from three sectors – industry, academia and government – to conduct research that has a direct impact on national security.
On average, LAS executes over $8 million in annual funding from the U.S. Department of Defense to leverage ideas and state-of-the-art research from academic and industry organizations to develop prototype solutions to the current and future challenges of the U.S. intelligence community.
In 2023, LAS sponsored research from seven companies and 12 academic institutions, with 23 faculty participants from institutions both within North Carolina and around the world. Each LAS principal investigator fully funds at least one graduate or undergraduate research student, with an average of 20 graduate students fully funded by LAS at NC State each year.