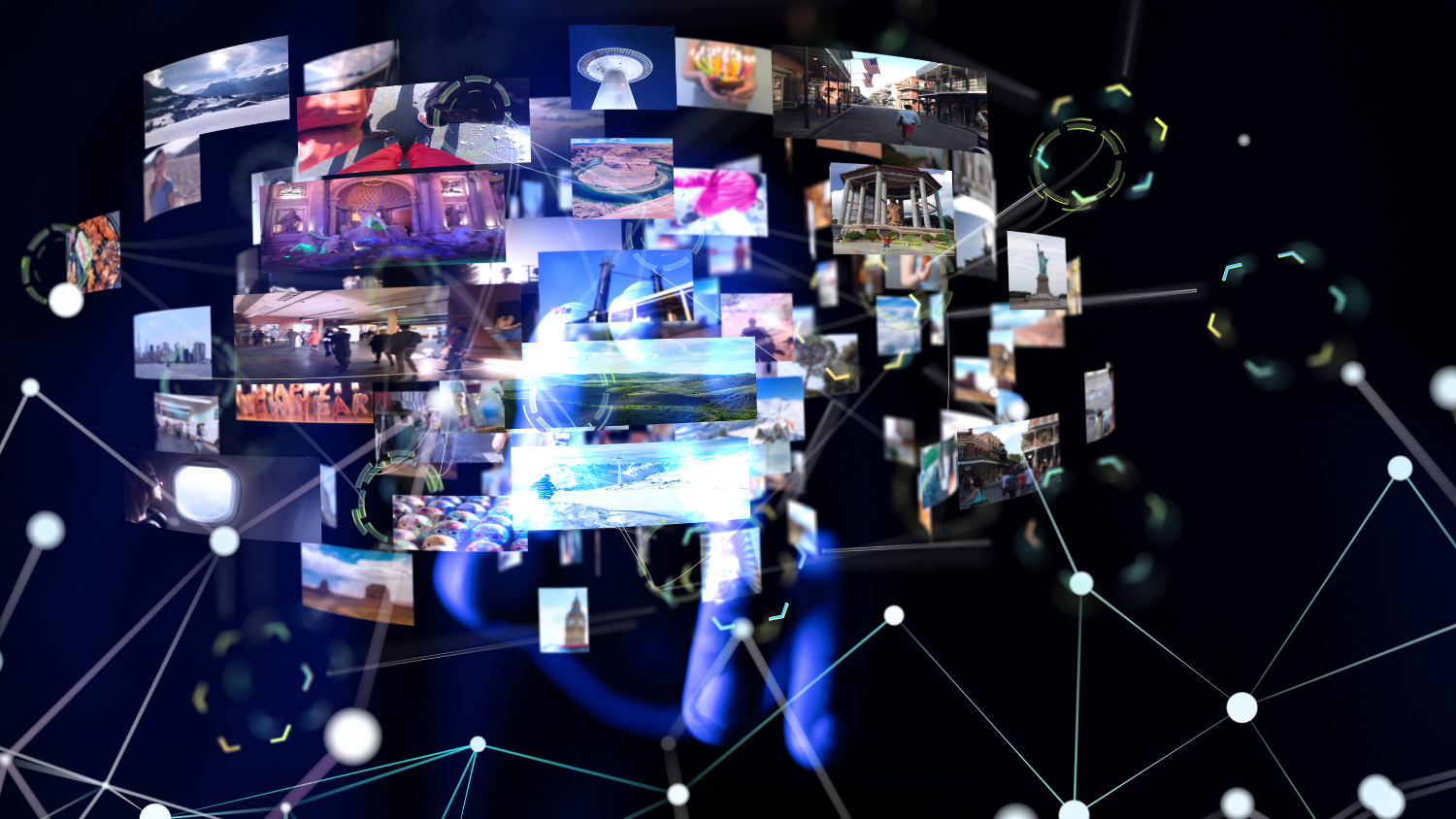
Impact Report
2024
Message from LAS Leadership
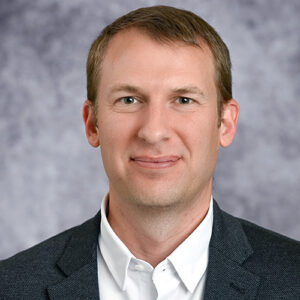
This past year saw LAS continue to innovate in our mission to engage with the broader analytics and research community to explore novel capabilities that impact intelligence analysis. The projects highlighted in this report serve both as a record of the accomplishments made by our staff and collaborators in 2024 and as a testament to the ways that LAS’s working model leads to impactful results. Close collaboration with end-users and operational stakeholders clarified significant research questions in areas like multimodal LLMs, human-centered AI, and human cognitive behaviors. Rapid prototyping and iteration amongst LAS projects and activities like SCADS resulted in novel capabilities like those for ensuring the security of AI models, triage of audio content, and machine learning operations. Integration with and support for transition partners enabled operational impacts in areas such as video analysis, speech-to-text, and manipulated media. 2024 once again showed that the capabilities of automated analytics and artificial intelligence are evolving quickly and their potential to benefit intelligence analysis workflows remains high. NC State remains proud to partner with the U.S. Intelligence Community and all of our academic and industry collaborators, as LAS continues to drive analytics innovation and shape the landscape of intelligence analysis.
Matthew Schmidt, Ph.D.
Principal Investigator, LAS
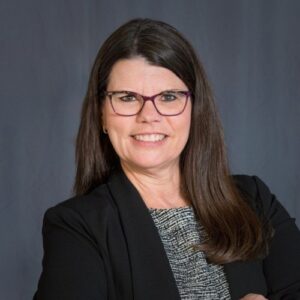
In 2024, LAS advanced intelligence analysis by integrating cutting-edge Artificial Intelligence and Machine Learning capabilities into analytic workflows. Our collaborations with the Department of Defense, NC State, and industry partners resulted in innovative tools that streamlined data processing, enhanced pattern recognition, and improved decision-making efficiency. These solutions empowered analysts to extract insights faster, reduce cognitive load, and focus on mission-critical challenges. Through research-driven development and real-world application, LAS delivered technologies that directly addressed analyst needs, from automating repetitive tasks to improving analytic transparency and trust. By bridging academic innovation with operational expertise, LAS continued to push the boundaries of intelligence analysis, ensuring analysts have the best tools to navigate an increasingly complex data environment. As we look ahead, LAS remains committed to driving the future of intelligence through the continued development of AI-enhanced analytic workflows.
Jacqueline Selig-Gumtow
Director, LAS
Our Mission
The Laboratory for Analytic Sciences is a partnership between the intelligence community and North Carolina State University that develops innovative technology and tradecraft to help solve mission-relevant problems. Founded in 2013 by the National Security Agency and NC State, each year LAS brings together collaborators from three sectors – industry, academia and government – to conduct research that has a direct impact on national security.
LAS By the Numbers
28 Collaborators
87 Students
~50 Full-Time Employees
35 Publications, Preprints, and Presentations
33 Prototypes, Datasets, and Models
56 Articles and Videos
Jump to:
Download:
Research Overview
Content Triage
Human-Machine Teaming
Operationalizing AI/ML
LAS research projects centered on three themes in 2024: Content Triage, Human-Machine Teaming, and Operationalizing AI/ML. At the year-end research symposium, LAS collaborators showed visitors from the U.S. Department of Defense how their recent work could alleviate real-world challenges faced by the intelligence community. Researchers presented lightning talks summarizing the year’s 34 projects, followed by poster sessions during which attendees could test out novel prototypes and confer with researchers.
Impact in Academia
Scholarly Publications
We’re proud to share the latest research articles published by our collaborators.
- John Wilcox, David R. Mandel, “Critical Review of the Analysis of Competing Hypotheses Technique: Lessons for the Intelligence Community,” Intelligence and National Security , Vol. 39, Iss. 6, 2024, pp. 941-962.
- Andrew C. Freeman, Ketan Mayer-Patel, Montek Singh, “Accelerated Event-Based Feature Detection and Compression for Surveillance Video Systems,” Proceedings of the 15th ACM Multimedia Systems Conference. Association for Computing Machinery, 2024, pp. 132-143.
- Andrew C. Freeman, “An Open Software Suite for Event-Based Video,” Proceedings of the 15th ACM Multimedia Systems Conference (MMSys ’24). Association for Computing Machinery, 2024, pp. 271-277.
- Eli Typhina, “Beyond Standard Practice: Tailoring Technology Transfer Practices Through Convergent Diagramming,” The Journal of Technology Transfer, 2024.
- Matthew Groh, “Evaluating Human Perception of AI-Generated Images Across Pose Complexities,” Proceedings of the 10th International Conference on Computational Social Science, 2024.
- Lucas Goncalves, D. Robinson, E. Richerson, Carlos Busso, “Bridging emotions across languages: Low rank adaptation for multilingual speech emotion recognition,” Proceedings of Interspeech, 2024, pp. 4688-4692.
- Abinay Reddy Naini, Lucas Goncalves, Mary A. Kohler, Donita Robinson, Elizabeth Richerson, Carlos Busso, “WHiSER: White House Tapes speech emotion recognition corpus,” Proceedings of Interspeech, 2024, pp. 1595-1599.
- Sunwoo Ha, Chaehun Lim, R. Jordan Crouser, Alvitta Ottley, “Confides: A Visual Analytics Solution for Automated Speech Recognition Analysis and Exploration,” 2024 IEEE Visualization and Visual Analytics (VIS), 2024, pp. 271-275.
- Hassan Shakil, Zeydy Ortiz, Grant C. Forbes, Jugal Kalita, “Utilizing GPT to Enhance Text Summarization: A Strategy to Minimize Hallucinations,” Procedia Computer Science, Vol. 244, 2024, pp. 238-247.
- Antonio E. Girona, James C. Peters, Wenyuan Wang, R. Jordan Crouser, “The Analyst’s Hierarchy of Needs: Grounded Design Principles for Tailored Intelligence Analysis Tools,” Analytics, Vol. 3, Iss. 4, 2024, pp. 406-424.
- Eric Xing, Pranavi Kolouju, Robert Pless, Abby Stylianou, Nathan Jacobs, “ConText-CIR: Learning from Concepts in Text for Composed Image Retrieval,” Computer Vision and Pattern Recognition 2025, 2024.
- Richard Lamb, “How textual features interact with cognitive factors during intelligence analyst information processing: Why environmental cognitive augmentation using artificial intelligence is an answer,” Computers in Human Behavior Journal, 2024.
- Negar Kamali, Karyn Nakamura, Aakriti Kumar, Angelos Chatzimparmpas, Jessica Hullman, Matthew Groh, “Characterizing Photorealism and Artifacts in Diffusion Model-Generated Images,” CHI Conference on Human Factors in Computing Systems, 2024.
Faculty Spotlight: Tim Menzies, Department of Computer Science, NC State University
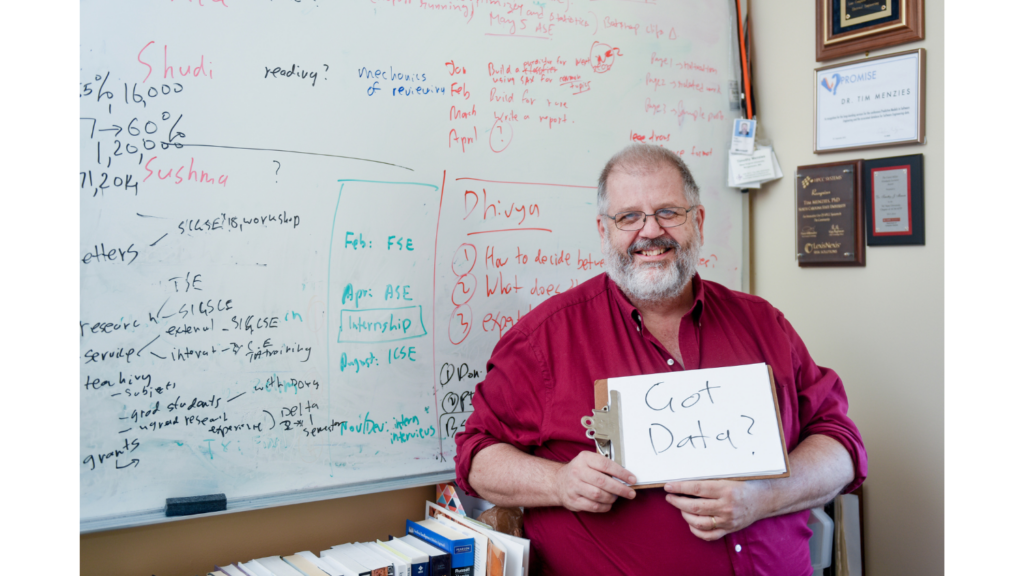
Since 2015, Tim Menzies, a professor of computer science at NC State, has been a key collaborator with the Laboratory for Analytic Sciences (LAS), tackling some of the most complex challenges in machine learning. His work with LAS has addressed critical issues that arise in the application of machine learning such as mitigating data drift, providing explainable results, and improving the efficiency of model training and inference.
Through this collaboration, Menzies and his students have had the opportunity to explore emerging ideas and refine them into meaningful, high-impact research. “Research needs much ‘watering of the seeds’ to grow good results,” he explains. “LAS funding let me work on preliminary ideas that grew into solid research presented at senior research forums.”
In 2024, Menzies led an LAS-sponsored project on next-generation prompt engineering, investigating how large language models (LLMs) can accelerate decision-making by serving as a “warm start” tool for sequential model optimization. The team’s findings demonstrated that across more than 20 problem domains, this approach significantly outperformed state-of-the-art incremental learning methods. Beyond the direct research outcomes, LAS has gained immeasurable value from its collaboration with Menzies. In recognition of his contributions to the field, Menzies was named an Association of Computing Machinery (ACM) Fellow in 2024—one of the highest honors in computing. His willingness to share his expertise and insight has benefited numerous LAS projects, and his technical discussions with staff have helped shape key research directions.
For Menzies, the benefits of this partnership extend to his students as well. His graduate students working on LAS projects gain firsthand experience in professional collaboration, learning the importance of responding to stakeholder needs. “It’s essential to understand LAS’s challenges and engage with them directly,” he advises his students and future LAS collaborators. “Your LAS contacts are not just sponsors—they’re research partners. Building those relationships is key.”
As LAS continues to push the boundaries of analytic sciences, partnerships like the one with Menzies exemplify the power of collaboration in advancing both academic research and mission-driven innovation.
A Better Image Search Tool
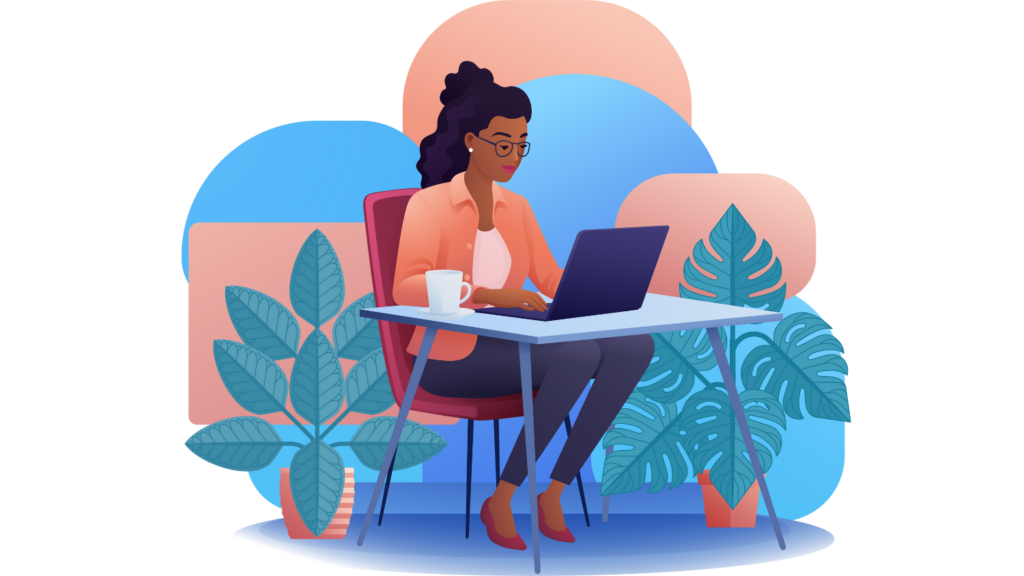
An LAS collaboration with faculty from George Washington University, St. Louis University, and Washington University in St. Louis focused on helping analysts use text-based descriptions to more rapidly find images relevant to their analyses.
A good image search tool helps investigators find the results they need when searching through scores of photographs, but it is often a challenge to get the search tool to understand what an analyst is looking for. In 2024, LAS partnered with a team of researchers from three universities to improve machine learning models used in text-guided image retrieval tasks: Robert Pless (Department of Computer Science, School of Engineering & Applied Science, George Washington University); Abby Stylianou (Department of Computer Science, School of Engineering and Science, St. Louis University); and Nathan Jacobs (McKelvey School of Engineering, Washington University in St. Louis).
In text-guided image retrieval tasks, an analyst uses ordinary language to specify what they want to find in a large set of images. The standard technique for training a machine learning model to do so is CLIP, or Contrastive Language-Image Pretraining. CLIP models are trained to have aligned text and image representations, so that, for example, the words “picture of a hotel room with two beds” and an actual photo of a hotel room have similar features recognizable by a computer. However, these models often struggle with complex, fine-grained queries that may occur in investigative tasks (e.g. “I’m looking for images of rooms with brown wallpaper that has vertical gold stripes with a flower motif embedded.”)
To train the models on complex text queries, the team also developed an LLM-based approach to generate complex captions that describe the notable differences between visually similar images. The team also developed a novel training loss for fine-tuning CLIP to support more linguistically complex queries by including sentence structure information in the training process. This allowed the researchers to develop a publicly available tool called “Will it Zero Shot?”, which seeks to determine if CLIP understands the concept you might be interested in well enough to do a search for it.
This work has had benefits for the university researchers outside of their collaboration with LAS, as well. They have been able to integrate and test these capabilities in an image search engine they deploy at the National Center for Missing and Exploited Children (NCMEC). This tool supports NCMEC analysts as they search for a hotel room seen in the background of sex-trafficking advertisements and aid law enforcement agencies in compiling evidence for prosecution.
Faculty Spotlight: Richard Lamb, Neurocognition Science Laboratory at the University of Georgia
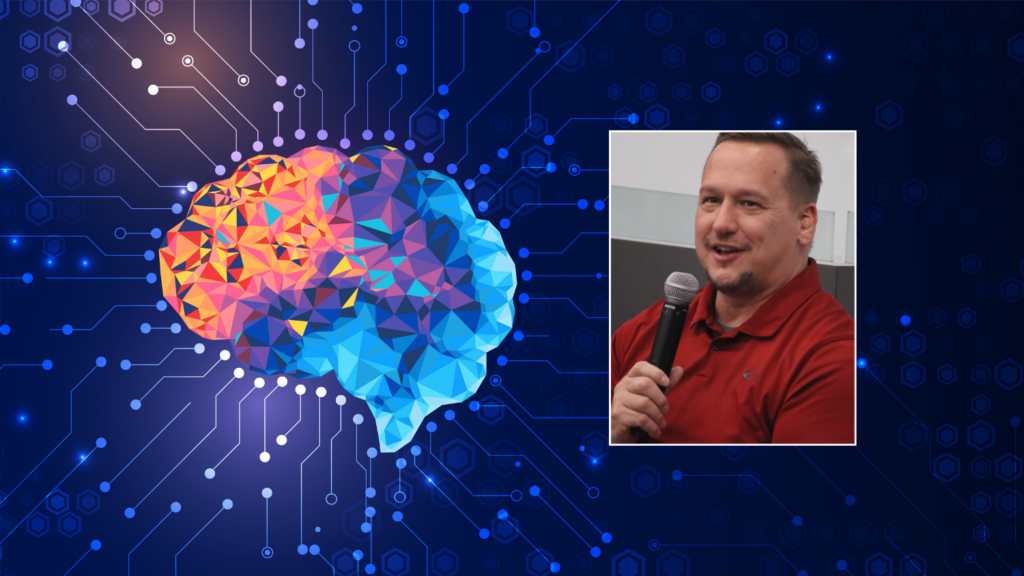
LAS collaborator Richard Lamb translates basic neuroscience into educational practices that improve learning outcomes and human information processing capabilities through cognitive augmentation. The opportunity to conduct research that enhances U.S. capabilities in new technologies and provides solutions to real-world mission-oriented problems prompted him to submit a white paper to LAS. Now, with support from LAS, he’s been focusing on integrating machine learning and artificial intelligence into neurocognitive research.
In 2024, he partnered with LAS as a year-long researcher and participated in the lab’s 8-week Summer Conference on Applied Data Science (SCADS). Lamb says the partnership has helped expand his research scope, publish groundbreaking findings, and contribute to mission-oriented solutions.
“The team is always open to new ideas and approaches, making the research process exciting and dynamic,” Lamb says. For example, a brainstorming session with LAS researchers led to the development of a novel approach to data analysis, which allowed him to develop a project using machine learning to predict mental health treatment outcomes.
The interdisciplinary environment at LAS allowed Lamb to work alongside experts from various fields, leading to novel methodological approaches and scholarly publications. He credits LAS with fostering an environment that turns ambitious ideas into impactful results.
“The interactions with LAS and its team were crucial for advancing my research and achieving significant outcomes, such as the development of a new diagnostic tool for cognitive assessment.”
Impact from Industry
Summarizing Multi-Lingual Conversational Speech with AI
RTX BBN is working with LAS to build a summary dataset that analysts can customize.
In a world overflowing with digital conversations, intelligence analysts face a daunting task—sifting through endless hours of foreign language chatter to extract key insights. In 2024, Hemanth Kandula, a senior research engineer at RTX BBN, partnered with LAS by participating in the Summer Conference on Applied Data Science (SCADS). While there he attempted to tackle this challenge head-on and create a system to cut through the noise and deliver concise summaries of these types of speech conversations.
Kandula is part of a research team at RTX BBN that has deep roots in speech and AI technology and has developed solutions for both commercial and government customers. The RTX BBN team had been interested in collaborating with LAS to build a cross-lingual speech summarization system for classified applications. LAS liked the concept and suggested Kandula come to SCADS to explore the idea further and see how it might provide value to analysts in practice.
At SCADS, Kandula, with support from his colleagues at RTX BBN, worked with other SCADS attendees to build a dataset of conversation summaries and evaluate different AI capabilities. Creating the dataset was no easy task. Unlike structured written documents, spoken language is complex. Conversations jump between topics, are riddled with informal speech, and often lack clear context. By augmenting and leveraging the 1997 CALLHOME dataset, which contains thousands of hours of audio recordings of people who agreed to be recorded in exchange for free international phone calls, Kandula was able to get feedback directly from analysts as to the functional utility of a variety of information extraction, machine translation, and topic modeling capabilities.
“At SCADS, you can directly communicate with the end user and find out the problems they are facing,” Kandula says. “You can build a system more curated to them.”
By the end of the conference, this close collaboration with analysts led to the concept and demonstration of a cross-lingual conversational data summarization system that can tailor both the style and content of its summaries to the needs of individual analysts. Kandula and his team at RTX BBN are now working with LAS outside of SCADS to continue to develop and prototype this concept with the goal of transitioning this work to operational networks.
Kairos Research Helps LAS Study Human Trust in AI
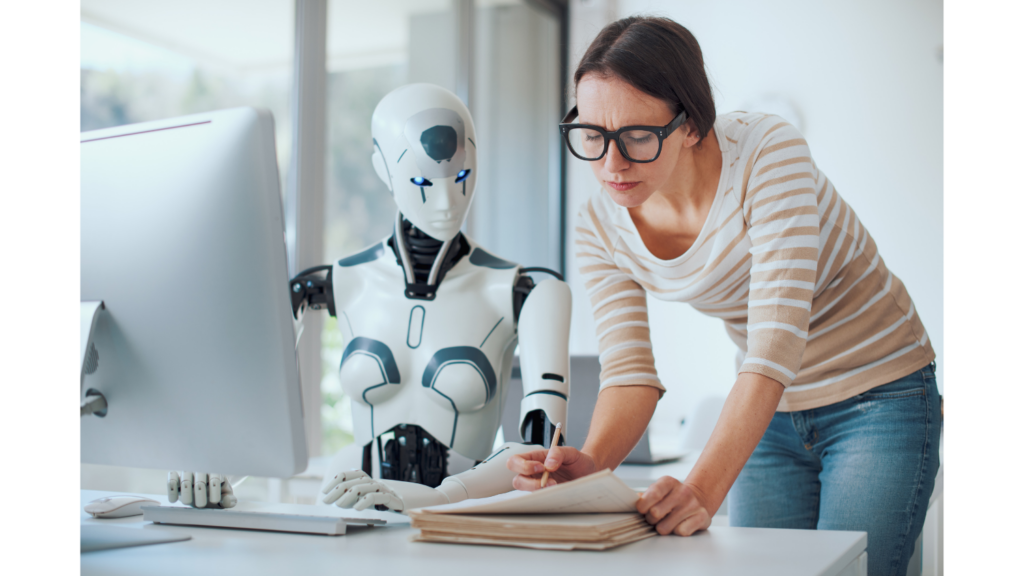
To investigate humans’ level of trust in their AI teammates, LAS collaborated with Kairos Research, a small business in Dayton, Ohio, that develops human-in-the-loop AI systems. In this study, participants were asked to complete an information triage activity using a synthetic email dataset, where they searched for specific information to answer questions. The team measured the impact of trust in artificial intelligence as the researchers varied the priorities of the AI teammate.
The results showed that trust in the AI was primarily driven by how well the AI completed the task, rather than how aligned its priorities were with those of human analysts. Interestingly, participants tended to overtrust the AI, even when it wasn’t performing optimally, which negatively affected their performance on tasks. The research team at Kairos is continuing to focus on whether repeated interactions with the same AI system can help analysts develop more calibrated trust—leading to more realistic trust and improved outcomes.
If users don’t trust the AI system, they may be more inclined to dismiss valuable results or accept results with errors, says Kairos researcher Cara Widmer.
“Understanding what information and experience an analyst needs to develop an appropriately calibrated trust in an automated system is essential.”
Impact on Capabilities
Refining Model Scanning Infrastructure with WOLFSCAN
The LAS model scanner project WOLFSCAN achieved significant milestones in 2024, establishing and refining a comprehensive model scanning infrastructure. The system streamlines the workflow for model security analysis and makes the results more accessible to stakeholders.
LAS software development staff implemented a preliminary scanning system incorporating multiple security checks, including malware detection software ClamAV for basic threat detection and AI security platform Protect.AI’s specialized model scanning capabilities. A cloud-based storage repository was established to store the models and their scanning results. It also has essential functionality for converting models to safe and unified formats to ensure broader compatibility and security. The scanner underwent a major architectural overhaul, introducing a more secure and scalable approach where individual scans are executed on temporary, isolated cloud-based virtual machine instances, preventing cross-contamination and ensuring resource isolation. This refactoring effort also included the implementation of a dedicated database for result storage and comprehensive model analysis capabilities, alongside establishing a controlled environment for dynamic analysis.
The software team developed a web application that provides a user-friendly interface for submitting and monitoring scan requests and viewing detailed reports of scanning results. The interface serves as a central hub for users to interact with the scanning service.
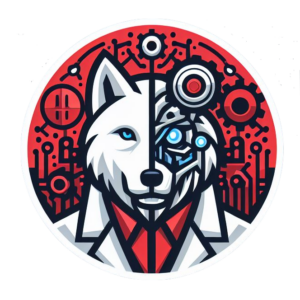
Enhancing Operator Comment Workflows
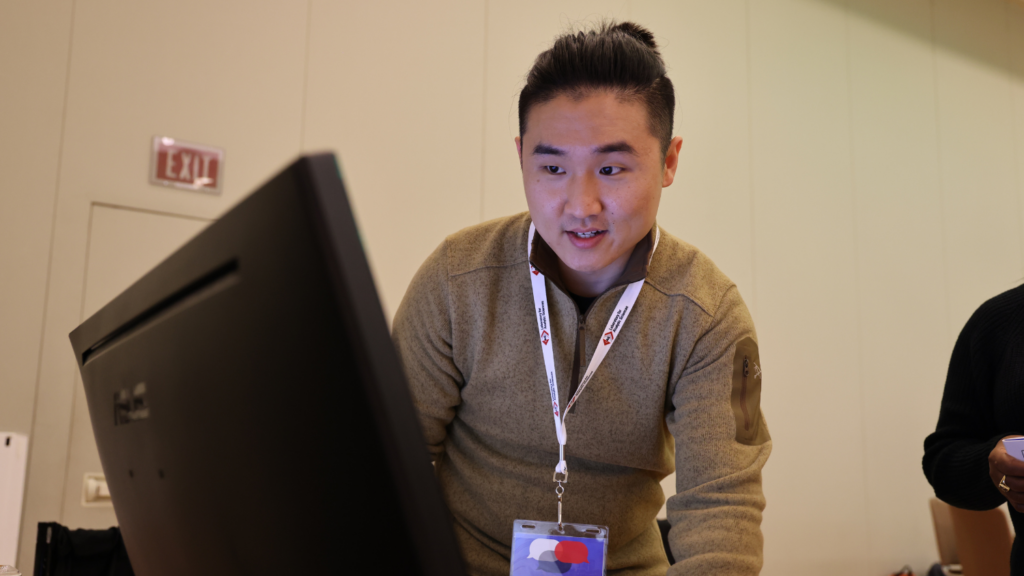
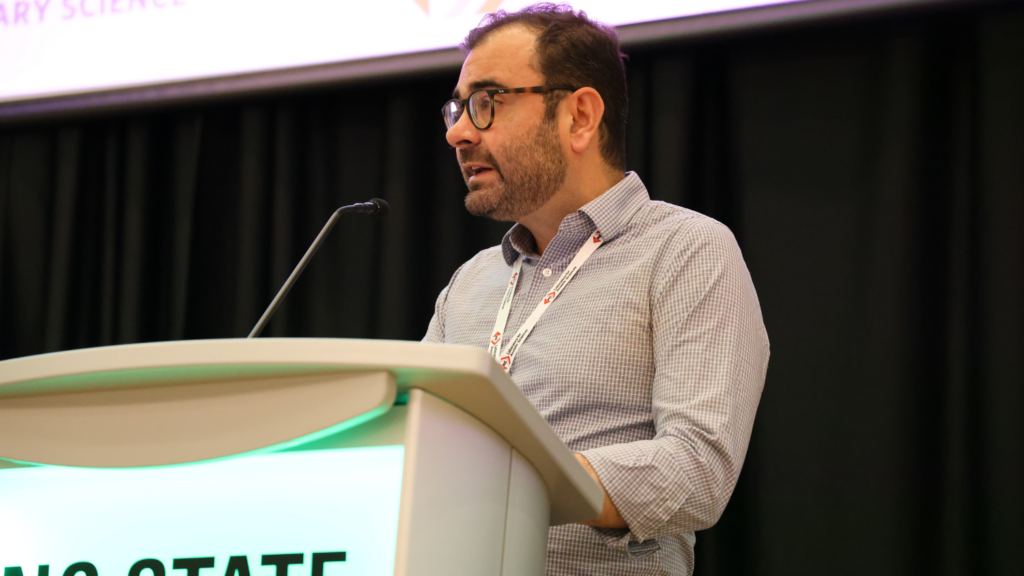
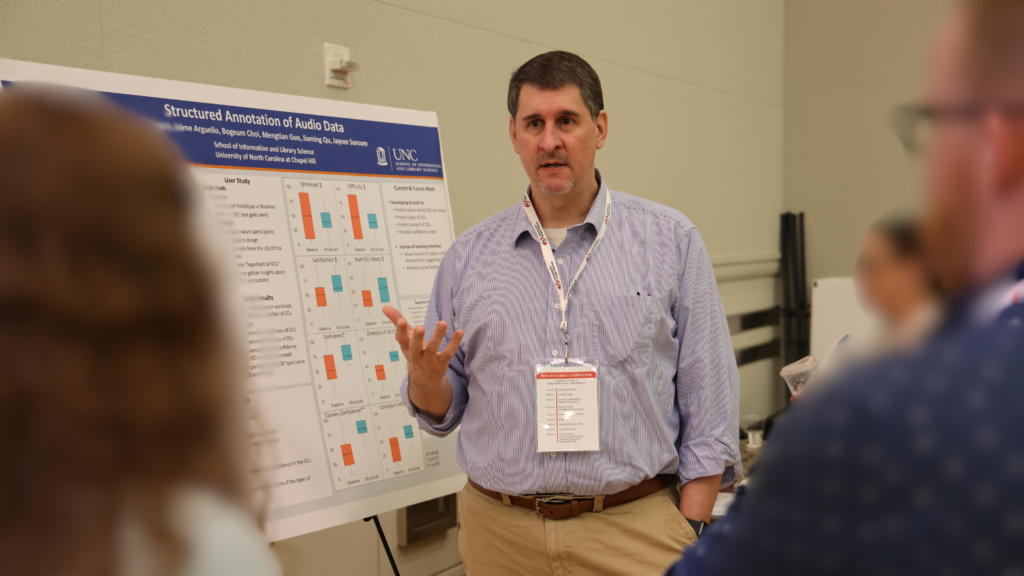
A collaboration with UNC-Chapel Hill helps language analysts listen to audio recordings, translate them into English, and add comments to help the reader understand who or what the speaker may be discussing.
In intelligence collection, there is often an abundance of implicit information that is understood by the foreign targets, but would not be easily understood by others. It is the responsibility of multi-disciplined language analysts (MDLAs) to make this information explicit in their translations. In other words, MDLAs listen to audio recordings, translate them into English, and add comments to help the reader understand who or what the speaker may be discussing. MDLAs typically achieve this by adding “operator comments” to their translations.
Making operator comments can be an ad-hoc and time-consuming process that requires MDLAs to decide when to make one, what information to include, and how to find that information. Once they make an operator comment in their transcript, the valuable information it contains will usually only be seen by the handful of other analysts who read the translation before it gets lost in a vast pool of unstructured data.
To improve this workflow, LAS collaborated with researchers at UNC School of Information and Library Science, led by Professors Rob Capra and Jaime Arguello. In 2022, UNC sought to understand the types of operator comments analysts make and the challenges they face when making them. Based on UNC’s findings the team developed a system, including a taxonomy of operator comments, to support analysts’ ability to make operator comments more efficiently and effectively.
In 2024, the National Security Agency’s Senior Language Authority (SLA) — a role responsible for end-to-end strategic planning for all civilian and military language analysts across the agency — incorporated aspects of UNC’s taxonomy into a proposed revision of the agency’s Language Analysis Quality Review policy, which is expected to be signed by the director of the National Security Agency in 2025.
Model Deployment Service Saves Time, Boosts Scalability
The LAS Model Deployment Service (MDS) is a system for deploying, scaling, and monitoring machine learning (ML) models that can be deployed across various cloud platforms, avoiding reliance on any single provider’s tools or service and ensuring better scalability and reliability. Utilizing Seldon Core V2 technology, often used by financial institutions to deploy models to assist with needs like fraud detection, MDS breaks model deployment into three parts: models, servers, and pipelines; and includes monitoring and metrics. It evaluates models by gathering metrics on user interactions, helping to identify issues and improve the system, and making it easier to move models to a corporate infrastructure.
LAS researchers working on the MDS project in 2024 aimed to simplify the process of deploying and evaluating ML models, making them more accessible and practical for researchers; and to evaluate solutions for scaling ML models to better shape the development of new production environments. Researchers had an opportunity to put MDS to the test at the 2024 LAS Summer Conference on Applied Data Science (SCADS). SCADS participant Chris Argenta of Rockfish Research built tailored daily report (TLDR) pipelines for people doing research experimentation when one or more of the pieces in the pipeline need to deploy ML models.
The use of MDS reduced the workload for researchers to run models, allowing them to devote more time to doing research. It also allowed researchers working on the TLDR pipelines to share custom pipeline modules instead of having testers run the models locally, thus, saving time and fostering the collaboration necessary to tackle the grand challenge of generating tailored daily TLDRs for knowledge workers.
Impact on NSA Initiatives
Advancing Surveillance Video Summarization
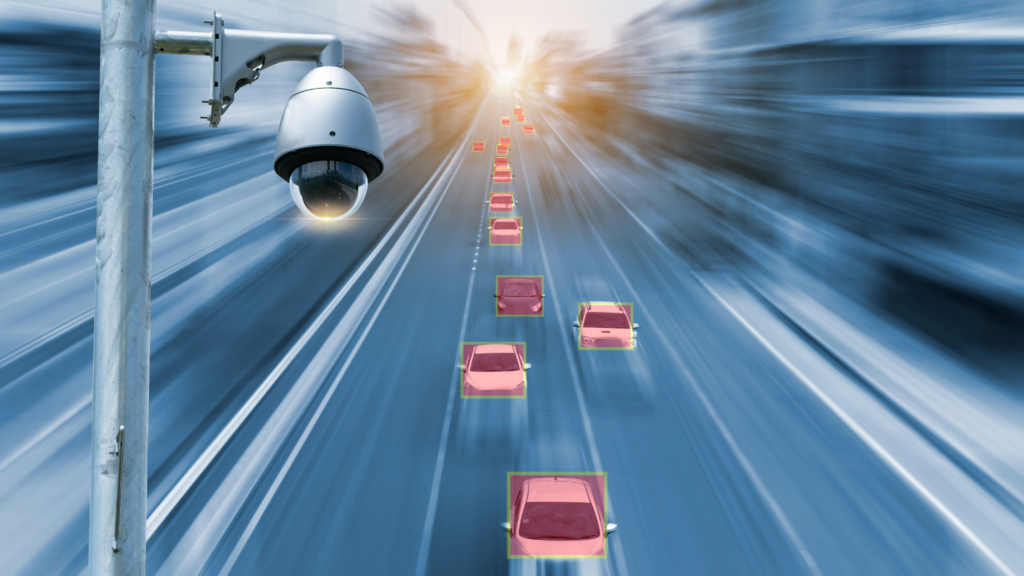
The EYEGLASS and SANDGLOW projects improve object recognition and activity detection, which are vital for situational awareness.
Originally conceived to advance analytical methods for dashcam video data, the EYEGLASS project strategically pivoted its focus toward the analysis of CCTV surveillance footage. Employing state-of-the-art machine learning and computer vision methodologies, the research team co-developed a sophisticated suite of modular Jupyter notebooks that provided a framework to build object recognition and activity detection machine learning models. The deliverables have been widely distributed to National Security Agency personnel, enabling the creation of tailored, mission-specific solutions within the do-it-yourself community.
The EYEGLASS project had a transformative impact on the agency’s capabilities and defense initiatives. Advancements in video analytics improve triage and situational awareness. By providing techniques throughout the triage process, this work directly strengthened national security objectives. Collaborative engagements with national laboratories, academic research institutions, and internal special projects have further amplified its influence, fostering a robust ecosystem of expertise and driving innovation through strategic partnerships. These alliances have extended the project’s operational reach and enriched its technical advancements.
A critical challenge addressed by this research is the stark disparity between publicly available datasets and the complexities of real-world video streams. By bridging this gap, the project ensures that analytics maintain high fidelity in operational environments. The implications are substantial: ineffective models risk either failing to identify critical threats or inundating analysts with false positives, thereby straining resources and compromising security operations. By delivering robust, field-tested solutions, this initiative fortifies mission-critical capabilities and reinforces the agency’s strategic objectives.
In video summarization, a prototype called SANDGLOW has advanced automated intelligence analysis of video content and object detection through the innovative application of multimodal language models (LLMs). The prototype was developed by LAS at the 2024 Summer Conference on Applied Data Science in conjunction with the NSA Texas Capabilities Directorate. It leverages cutting-edge technologies like Phi3.5-vision and Mistral-Nemo.
Developers on the research team tested SANDGLOW’s ability to summarize and analyze a diverse range of video scenarios accurately.
“From one-minute aviation clips to hour-long political analyses, SANDGLOW maintains remarkably high accuracy across varying lengths and complexities,” says LAS developer Paul N.
The team used popular car movies to test the prototype’s object detection capabilities. SANDGLOW provided detailed identifications, including vehicle make, model, color, passengers, and even visible license plate numbers, for example.
“The categorization abilities of the system allow rapid classification of video content, conserving computational resources and accelerating strategic decision-making processes,” Paul N. says.
Future iterations of SANDGLOW are poised to integrate state-of-the-art technologies, such as Pixtral and VLLM, to overcome current hardware limitations and enhance computational efficiency. SANDGLOW’s research team says these advancements promise greater scalability, accuracy, and responsiveness in high-stakes intelligence environments, underscoring SANDGLOW’s potential as a transformative solution in computer vision and automated intelligence analysis.
A Revolutionary Initiative to Strengthen Internet Integrity
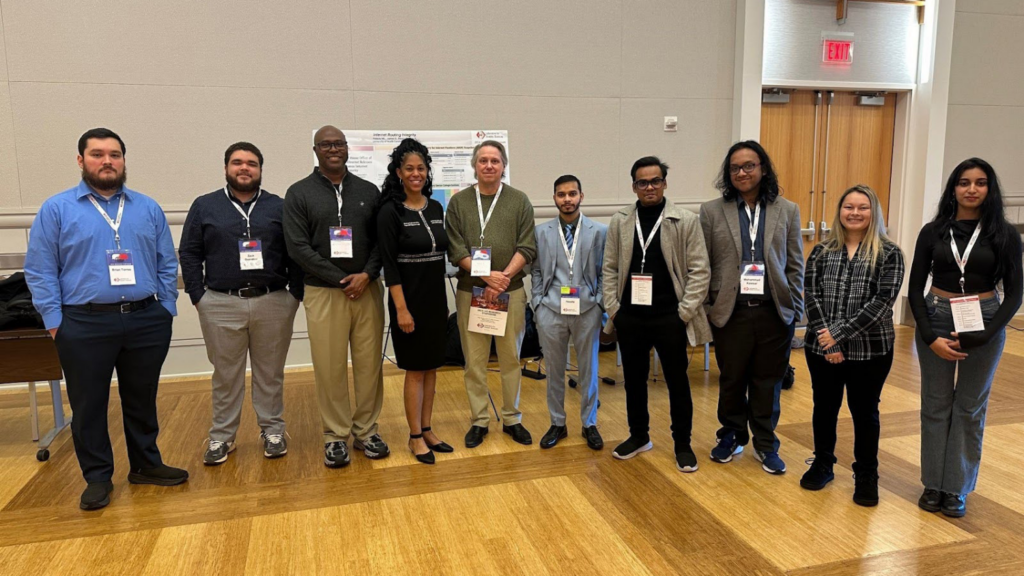
In an increasingly connected world, safeguarding our digital economy is crucial.
In 2024, LAS researchers partnered with the NSA’s Research Technical Director Neal Ziring and the University of North Carolina at Pembroke on an initiative to strengthen U.S. internet integrity. Recognized as a Center of Academic Excellence in Cyber Defense, UNC Pembroke provided the perfect foundation for this ambitious project, which harnessed cutting-edge artificial intelligence and machine learning technologies. Cybersecurity professor Prashanth BusiReddyGari and his senior design students at UNC Pembroke worked alongside the team to develop an advanced system to address vulnerabilities in internet routing infrastructure. The project successfully advanced routing security by incorporating Resource Public Key Infrastructure (RPKI) and Route Origin Authorization (ROA) protocols, using AI models to identify unauthorized routes with precision and scalability. This innovative approach mitigated potential disruptions and security risks stemming from routing anomalies, marking a significant advancement in protecting global internet infrastructure.
The stakes couldn’t be higher, says Felecia ML., one of the LAS researchers involved in the project.
“In an increasingly connected world, the integrity of internet routing isn’t just about protecting individual users—it’s about safeguarding our entire digital economy.”
The team developed a custom-built platform that seamlessly integrated bulk Whois data from the American Registry for Internet Numbers (ARIN) with advanced RPKI validation tools to process and validate over one million routes across U.S. networks. Leveraging cutting-edge AI techniques like Sentence Transformer and ChatGPT, the team categorized more than three million ARIN-registered organizations into the 16 sectors defined by the Cybersecurity and Infrastructure Security Agency, enabling precise identification of sector-specific vulnerabilities. Supported by a MongoDB-backed framework, the system efficiently stored and organized current and historical internet routing data, which was visualized through interactive dashboards to track RPKI adoption trends over time. The project earned recognition when presented to the National Institute of Standards and Technology, offering transformative solutions for addressing critical challenges in securing the internet.
“This effort showcased the critical role of academic partnerships in advancing cybersecurity to secure vital networks,” says Felecia ML.
Impact to Mission
Enhancing Speech-to-Text Triage with GUESS
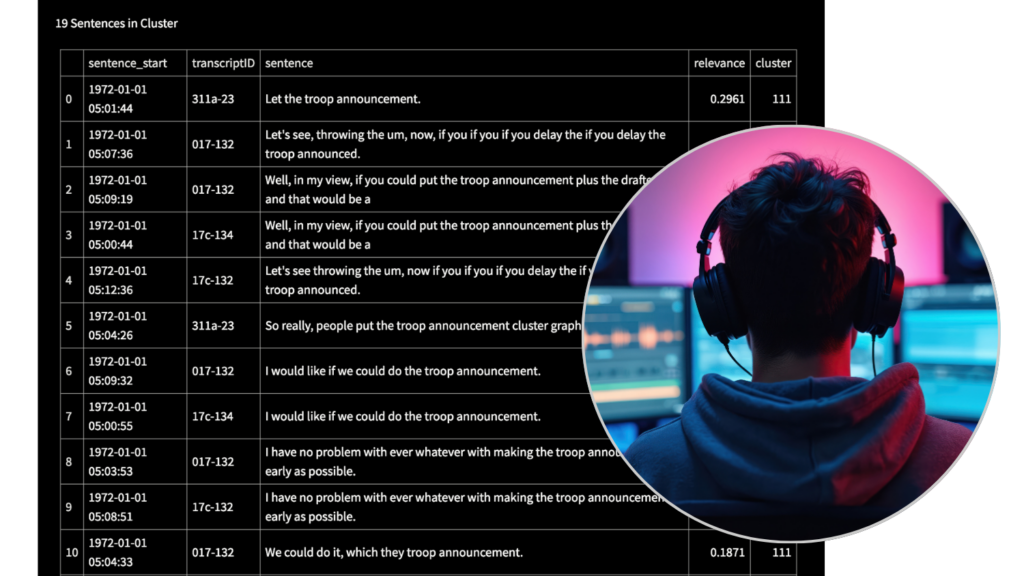
LAS developed a prototype that searches audio transcripts.
Analyzing audio data in the intelligence community presents unique challenges, especially when the challenging data environment hurts speech-to-text (STT) transcription quality. Traditional keyword search methods often fall short, forcing analysts to manually sift through audio to find relevant content—a time-consuming and exhausting process. To address this, the Laboratory for Analytic Sciences (LAS) developed GUESS (Gathering Unstructured Evidence with Semantic Search), a prototype that enables semantic search and iterative refinement to make STT triage more efficient and effective.
In early 2024, LAS hosted a week-long focused-discovery activity (FDA) where five Department of Defense analysts tested GUESS against their existing triage methods. The results were decisive: analysts found key information significantly faster, with greater accuracy, and with far less fatigue. They also reported that GUESS preserved their sense of control over the process, reinforcing confidence in AI-assisted search. By the end of the FDA, participants were eager to integrate the tool into their workflows.
The success of GUESS didn’t stop with the FDA. To support broader adoption, LAS created a “how-to” guide detailing best practices for using GUESS effectively in analyst workflows. In 2024, the guide was accessed by 862 analysts from 403 organizations, with 352 returning users from 207 organizations, reflecting sustained interest.
Perhaps the most significant outcome of this work is its influence on future AI-enabled analytic tools. The core concepts behind GUESS—semantic search, iterative refinement, and human-machine teaming—are now driving the development of a new corporate capability set to launch in 2025. Funded under an ODNI pilot program focused on AI-assisted workflows, this effort will explore how semantic search will impact workflows beyond the confines of an FDA.
By proving the value of human-AI collaboration in real-world intelligence analysis, GUESS has laid the groundwork for lasting improvements in STT triage. As these innovations move from prototype to operational tools, they will help analysts spend less time searching and more time extracting meaningful insights—a crucial step forward in optimizing analytic tradecraft.
Intelligence Analysts Trained to Recognize Manipulated Media
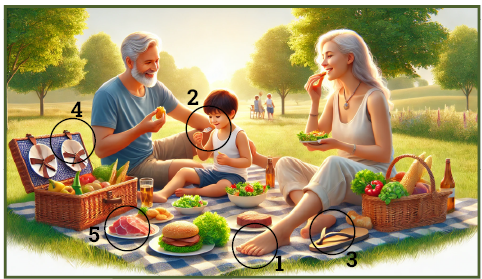
Northwestern University and LAS produced a how-to guide to help analysts tell the difference between AI-generated and real images.
Generative AI’s proliferation across modalities and its ability to produce synthetic media that mirrors real content means that it is increasingly likely that intelligence analysts will encounter AI-generated content within their analytic workflows. For intelligence analysts to produce sound analysis from source materials (and adhere to ODNI’s Intelligence Community Directive 203 – Analytic Standards), they will need support in recognizing and understanding generated media. In other words, analysts must determine the reliability and credibility of the source, whether there is any reason to doubt the authenticity of the media, plausible alternatives for the use of generated or manipulated media, the relevance of the media, and more.
To explore what characteristics analysts should consider when scrutinizing image content, LAS collaborated with Northwestern University’s Matt Groh, an assistant professor in the Management and Organizations department at the Kellogg School of Management. Groh and his team developed “a taxonomy for characterizing signs that distinguish between real and diffusion model-generated images.”
The team trained dozens of analysts to test the taxonomy’s effectiveness in enhancing the analysts’ ability to identify AI-generated content. In the training, participants were asked to review a dynamic set of real and synthetic images, once before they received training and again afterwards. In the first round (before training), analysts correctly identified 70% of the images as real or synthetic. In the second round (after training), analysts’ ability to discern AI-generated content from real jumped to approximately 77% correct – a statistically significant increase. Several analysts also noted that they could immediately apply the training to their work and had an enhanced ability to identify between authentic and synthetic images. The success of the training spurred the NSA’s Senior Intelligence Analysis Authority and the Senior Data Science Authority to investigate ways to make the training available to a broader set of intelligence analysts.
Crucial to the partnership and work in 2024 was LAS’s engagement with Candice Gerstner, co-chair of ODNI’s Multimedia Authentication Steering Committee. Gerstner helped steer the research to align with existing training offered across the intelligence community. LAS also partnered with SIAA, which sets the strategy for the next generation of the intelligence analyst workforce, and other key stakeholders that inform analyst training and tradecraft.
‘It’s Classified’ – But Here’s the Unclassified Summary
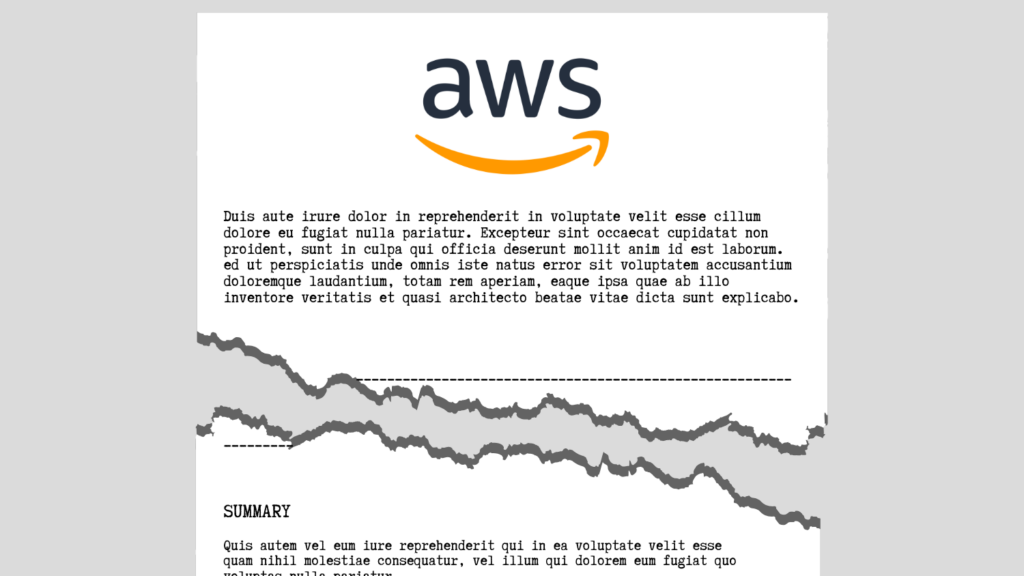
Amazon Web Services and LAS used unclassified datasets to simulate automated tearlines that summarize classified reports for a broad audience.
For an intelligence report to be useful, it’s essential that the report can be shared with the right customers. This can be challenging since not all customers have the same clearance level or access to information. So how can an analyst make a report widely available when the report may be highly classified or controlled? In theory, the answer’s simple – just write a “tearline,” or a summary that provides the same key findings but isn’t as controlled. In reality, though, the time and cost required to generate tearlines can often outweigh their anticipated benefit, meaning fewer tearlines get created than ideally should be. In collaboration with the Amazon Web Services (AWS) Professional Services team, LAS is exploring the integration of machine learning technologies into corporate intelligence workflows to address this gap and streamline tearline creation.
The generation of a tearline is guided by a set of rules that ensure the content is at the appropriate classification level and does not reveal sensitive sources or methods. While many of these rules are explicitly defined in reporting guidance and secure classification guides, some are implicit, often learned from experience, and unique to each office. The AWS team developed a large language model-based pipeline to codify these unwritten rules using report-tearline document pairs. LLMs can use these rules to drive the production of higher-quality tearlines, and analysts can use them to infer tradecraft.
LAS identified an unclassified proxy dataset from biomedical journals PLOS and eLife. It provides lay summaries alongside complex scientific abstracts. These document pairs mimic the relationship between classified reports and tearlines, serving as training data for machine learning models.
Consider the following proxy example. Researchers tell the model, “Do not include the scientific name of a cell or cell type, but instead refer to the cell in layman’s terms. Instead of ‘alveolar macrophages’, say ‘lung cells’ …” Instructing the AI to focus on the omission or generalization of technical (or classified) details helps write summaries (or tearlines) that could be shared with a wider audience.
The AWS team developed a custom LLM-based evaluation suite to assess rule-following ability, similarity to ground truth, and overall summary quality. Using the proxy dataset containing lay summaries of technical papers, they compared generation quality of various foundation models, model parameters and prompting techniques. They also focused on building analyst trust in the generated tearlines by developing explainability techniques like providing in-line citations of the rules used to write the tearline. These results will inform a follow-on effort in 2025 to prototype a tearline generation capability in the corporate reporting test system.
Summer Conference on Applied Data Science
SCADS researchers focused on mission-relevant capabilities in automated text summarization, user experiences, and tailored recommendations.
5 End-to-End Prototypes
35 Project Reports
4 Synthetic Data Sets
Partnerships
Meet the 2024 Collaborators
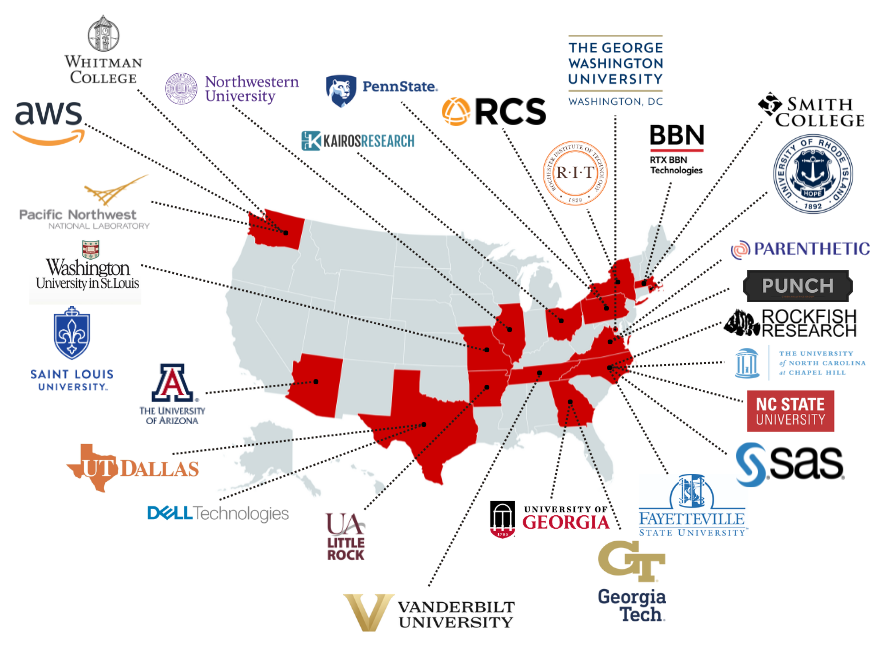
SAS and LAS Develop Tools for Non-Technical Analysts
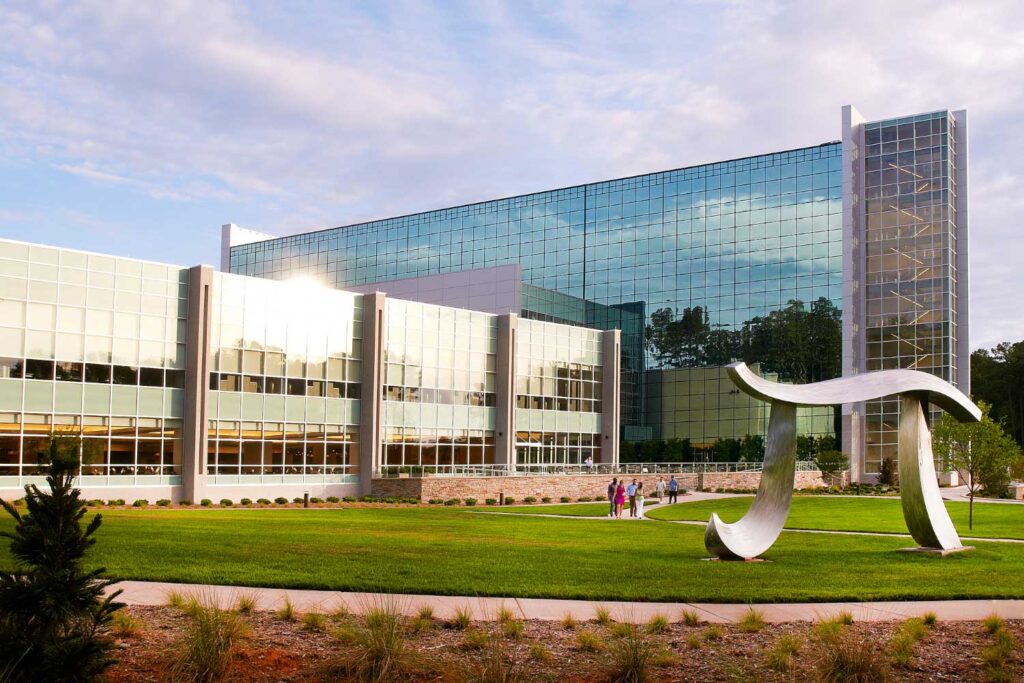
Headquartered in Cary, North Carolina, SAS Institute maintained its long-standing collaboration with LAS in 2024. SAS, a premier provider of data analytic solutions, played a pivotal role in innovating the triage process for technical intelligence, which directly bolstered a less visible, yet vital, mission for LAS stakeholders.
In 2024, researchers from SAS worked with LAS to develop workflows and tools that allow non-technical analysts to triage, sort, summarize, and curate specific technical details from a large body of unstructured, open-source information. This could be anything ranging from how-to guides to patent applications to research papers.
For example, an analyst could have a group of images of circuit boards, but they don’t have the technical background to know if the circuit boards are from toaster ovens or GPS receivers. The automated system developed by the 2024 project can sort through a set of circuit board user manuals and technical documents to provide context for the analyst and help them determine if the images are relevant to their interests.
LAS’s long-standing collaboration with SAS Institute has proven valuable, not only delivering immediate analytical benefits but also facilitating the transfer of critical technical insights. Ongoing dialogue and technical exchanges with SAS enabled LAS to proactively investigate state-of-the-art data analytics methodologies driving innovation in upcoming projects, including, notably, a workflow metrics and performance evaluation effort to be undertaken in 2025.
Pacific Northwest National Lab Collaborates with LAS
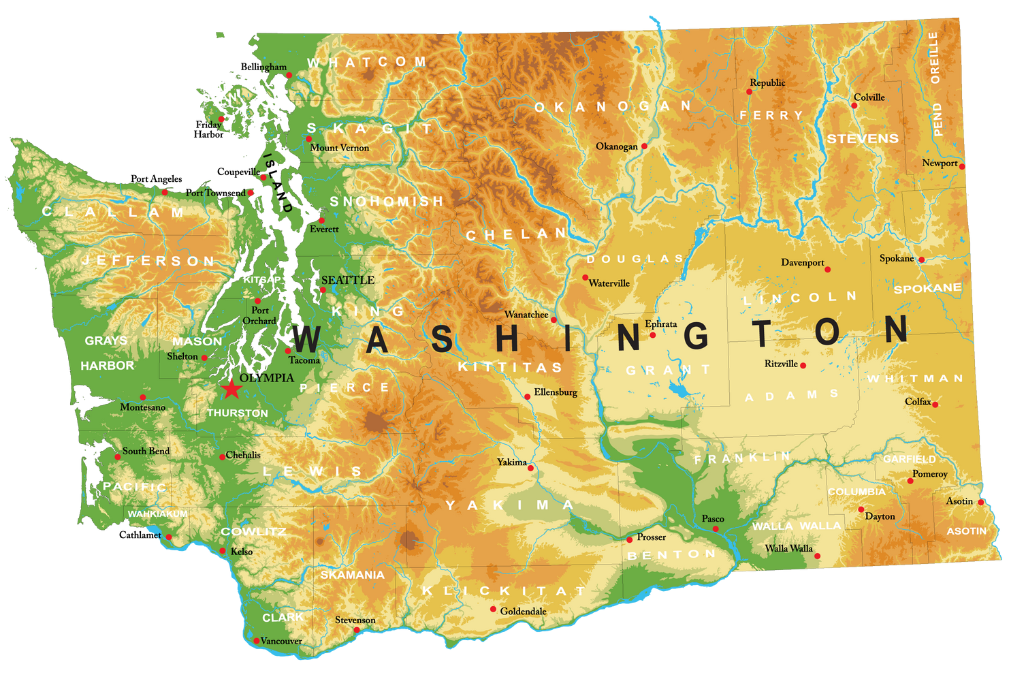
Since 2015, Pacific Northwest National Laboratory (PNNL) has been an active collaborator and partner with LAS. With a strong focus on leveraging subject matter expertise across technical domains, PNNL’s integration with the LAS research mission supports a variety of opportunities for cross-organizational staff engagements. Seminar series talks, immersive workshops, and project-specific collaborations continue to support the growth of this unique partnership with the Department of Energy. Collaboration highlights from 2024 include:
Summer Conference on Applied Data Science. In 2024, three PNNL staff traveled to North Carolina to spend a week in person with the attendees and get the unique chance to work directly with people from the intelligence community. As visitors, PNNL staff get to see how their research is being used and how it’s contributing to the mission.
“I loved being at SCADS,” said Michelle Dowling, a data scientist at PNNL. “It was an amazing experience and I walked away with lots of useful ideas and feeling excited to tell others at PNNL about them!”
Research Project on Knowledge Graphs via Conformal Prediction. Karl Pazdernik is a chief data scientist at PNNL. He also holds a joint appointment as a research assistant professor at NC State in the statistics department, conducting data analytics and national security research. Through his faculty appointment at NC State, Pazdernik and his colleague in NC State’s statistics department, Srijan Sengupta, led an LAS project to help intelligence analysts more objectively assess how reliable a knowledge graph is.
Lab Technical Research Exchange. LAS hosts a monthly, unclassified, seminar to bring together professionals, enthusiasts, and curious minds to learn about advancements in artificial intelligence, machine learning and data science. In 2024, each session featured two distinguished speakers from US National Laboratories or federally funded research and development centers (FFRDCs). PNNL’s presence at the LAS provided additional insight into technical/research topics of interest to the community. As a result, PNNL staff have kicked off numerous technical exchange meetings with staff across the Department of Energy complex and established close connections with LAS researchers and analysts.
Student Engagement
Computer Science Students Lay the Groundwork for Future Advances in Audio Sensemaking
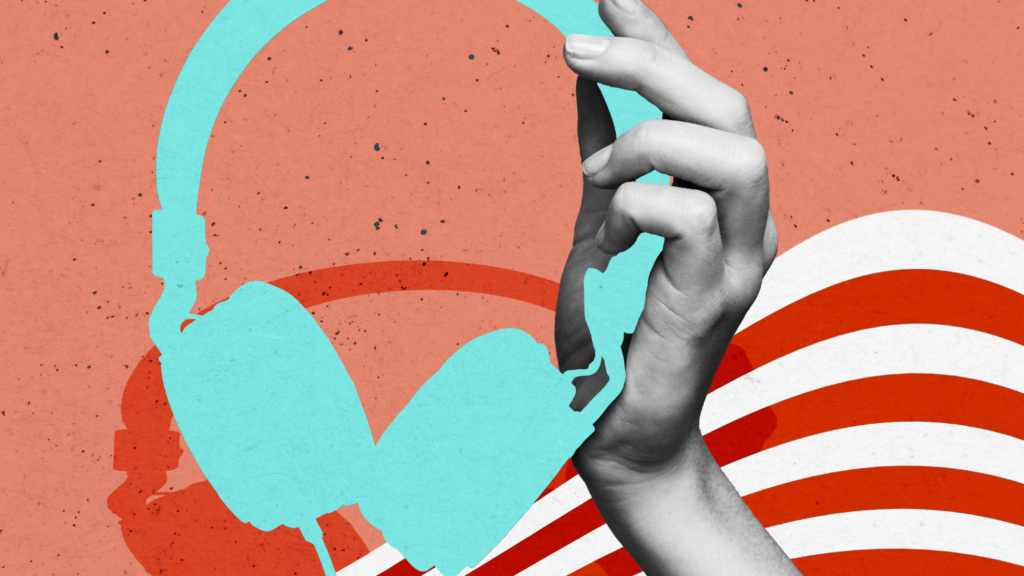
Analyzing audio data in the intelligence community presents unique challenges. To better understand and improve speech-to-text (STT)-based workflows, the Laboratory for Analytic Sciences (LAS) partnered with a North Carolina State University Senior Design team in 2024 to develop granular instrumentation capabilities for STT applications. By capturing detailed data on how analysts interact with STT search and navigation tools, this project lays the groundwork for more efficient and effective audio sensemaking workflows.
This effort is part of LAS’s long-standing partnership with NC State’s Computer Science Senior Design Center, one of 25 Senior Design projects LAS has sponsored since 2016. More than 100 students have worked on real-world technical challenges relevant to national security through these collaborations. These projects provide students with hands-on experience while generating valuable technical innovations that support intelligence mission needs.
For this project, the Senior Design team focused on capturing detailed metrics of how analysts engage with STT data. The team designed a custom JavaScript instrumentation library that integrates with LAS’s Common Analytic Platform (CAP), a research environment for developing and testing analytic tools. This new capability tracks key STT interactions identified in past human-centered research conducted at LAS.
The collected data is stored in a SQL-based database for future visualization and analysis, allowing LAS researchers to evaluate how analysts use STT in real-world scenarios. These insights could inform decisions on STT algorithm improvements, targeted deployments of alternative STT models, and UI optimizations for intelligence workflows.
With a strong foundation built by the student team, this project is set to make a meaningful impact. LAS plans to transition these instrumentation capabilities to the corporate network in 2025, where they will directly support new initiatives in audio sensemaking. By integrating analyst workflow data into operational systems, LAS can help the intelligence community make data-driven decisions on STT investments and optimize tools for real-world missions. While the immediate impact may be small, the long-term potential is significant—refining analytic tradecraft, improving STT-based search and discovery, and driving innovation at the intersection of AI and human-centered analysis.
Staff
Meet the Team
Staff Spotlight
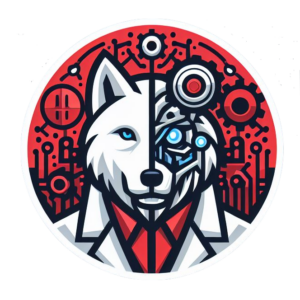
Share your story.
We are looking for stories about the impact of our work for our annual LAS Impact Report. If you have received an award, published an article, developed a product, applied research to real-world challenges, joined a board, or otherwise made an impact through your work with LAS, let us know with this form.