Sight Unseen, Students Design UX for Intelligence Analysts
NC State College of Design students tackled real-world problems and created user interfaces for intelligence analysts. The project demonstrates the power of academia-government partnerships in safeguarding national security.
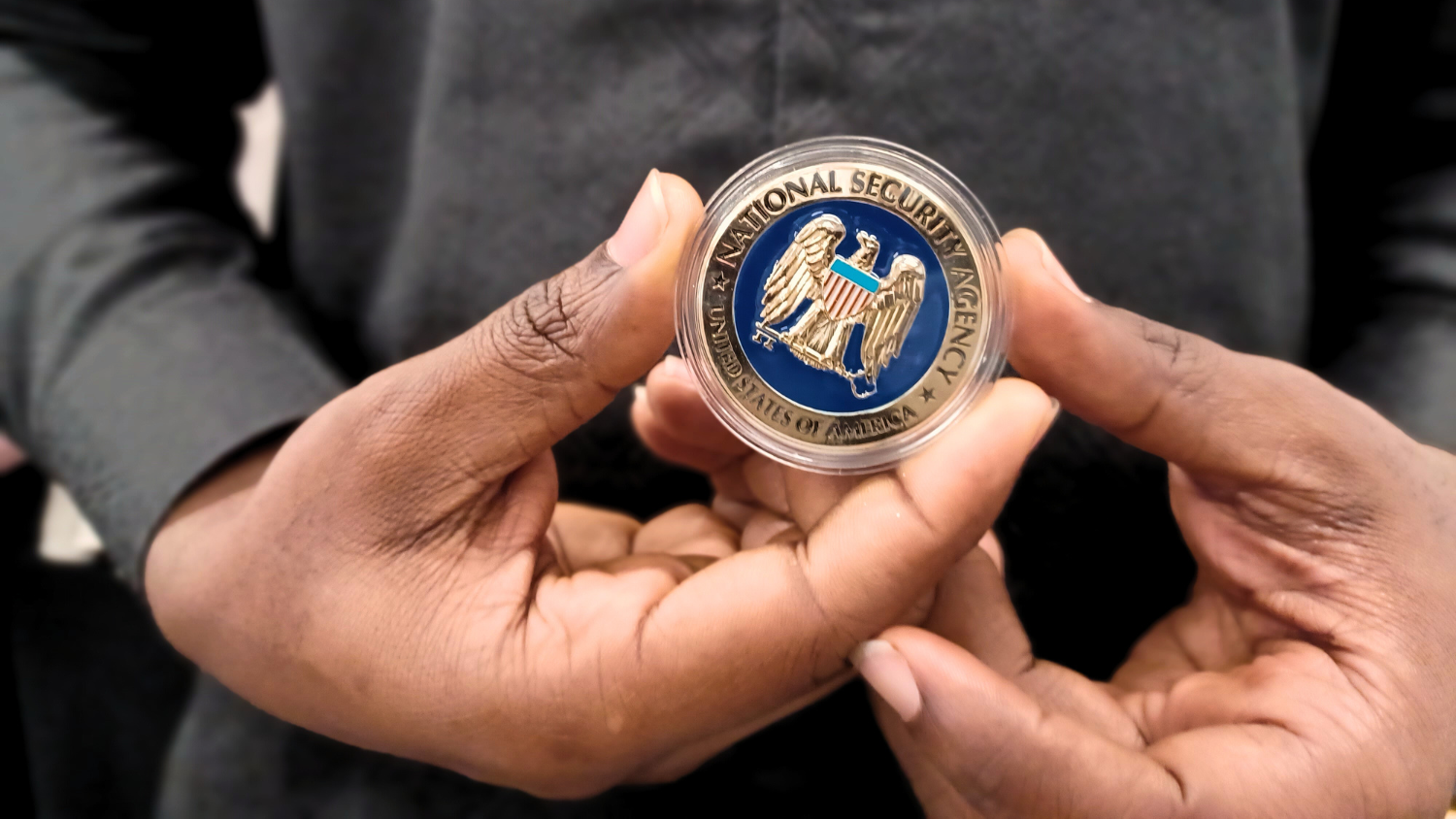
“I was fascinated by the concept of working with students to visualize potential solutions to the many pain points we face as analysts.” – Tim S., LAS intelligence analyst
A Fictitious Terrorist Attack
“In 2019 a bombing killed two people and injured others in the city of Macondo, a mountain town in Oceania. A commercially made drone fitted with an IED (improvised exploding device) targeted a coffee shop on North Duke Street in the morning hours of April 10, 2019.”
This fictitious scenario is one of many that our nation’s intelligence analysts might face. This year the Laboratory for Analytic Sciences worked with Professor Helen Armstrong’s students in the Master of Graphic & Experience Design (MGXD) program at the NC State College of Design. Their assignment? To consider how analysts at different stages of their careers might use particular workflows, or particular analyst challenges, to investigate this scenario and design an interface that could address those challenges.
Developing Interface Concepts for Different Analyst Workflows
The intelligence analysts responsible for finding and making sense of the technical data that will be used to inform the country’s decision-makers, via a final intelligence analysis report, are known as target digital network analysts (TDNAs). For this semester’s work, the students were separated into four groups. Each group played the part of a TDNA facing different analyst challenges, with various skill levels and expertise, and with differing tasks to complete.
While the students were eager to start the assignment, LAS wanted to ensure they had the tools necessary to tackle this complicated task. Led by Professor Helen Armstrong, the graduate students were divided into four groups and given a persona along with a fictional scenario. Students also interviewed real-world intelligence analysts to understand how these pain points affect daily workflows. Taking this information, they set about their work via user experience (UX) design methods to research and develop innovative solutions that addressed user pain points and unique design criteria. LAS mentors met with students multiple times both virtually and in person to answer questions and to give feedback on prototypes created.
The Final Product: Four Prototypes
The four student groups each developed a prototype they presented to LAS at the end of the semester.
Hello, World!
DataTrace for Susie, an entry-level analyst on her first day
This fictional scenario describes a rookie analyst’s first query. Students designed a high-fidelity UX prototype they named DataTrace, based on “Susie,” an entry-level analyst persona supplied by LAS. Susie has been tasked with looking up an email address that has recently been in communication with a terrorist group from the fictional country Zendia. Susie’s team believes it belongs to a new operative.
She opens the DataTrace interface and types into the search field.
“I am a new network analyst and need help querying an email address that we suspect is being used by the Zendian terrorist organization in relation to a bombing in the Oceania city of Macondo.”
Because DataTrace was designed to address the anxieties of a new analyst, Susie finds the natural language approach to queries very intuitive.
At the end of the day, Susie has run several queries and analyzed a range of data. She is ready to review her activity and submit an analysis summary to her mentor. She expands the data basket where she’s been dragging and dropping relevant information for the AI to synthesize in the background. The data basket has two tabs.
The first tab highlights her activity throughout the day. She can share that with her mentor and refer back to it the next day as a reminder of where she’s left off. The system pulls out specific details such as the resources she’s accessed, the queries she’s run, and the results of her analysis.
On the second tab, the system automatically summarizes those results using natural language processing. The system points out areas that were not covered by Susie’s analysis such as detailed content analysis and an insufficient understanding of IP addresses at the bottom of the page. The AI toggles on filters to either suggest areas of improvement or expand the summary based on the information Susie dropped into the data basket. She has the option to edit or regenerate the summary, but she likes the way the system summarized her work and made it easy to share it with her mentor to review her work. She believes her analysis will help her contribute to her team’s mission.
- Watch a video of the proposed DataTrace interface, designed by Kweku Baidoo, Alexis Boone, and Rebecca Planchart: https://tae.ncsu-las.net/Susie
Mind The Gap
RedFlag for Josh, a junior analyst who is struggling to understand when his data sources have gaps
This scenario describes how an analyst with more intermediate experience determines what data they are missing. RedFlag is a high-fidelity UI/UX prototype created by the second team of students. It’s based on the fictional analyst persona “Josh,” a junior analyst with a little more experience than Susie.
Josh begins his day by resuming the active investigation into the bombing in Macondo. In RedFlag’s main panel, he reviews a notification he filed last Friday alerting him that the data from the IED drone’s RFID chip is corrupted. Josh quickly examines the notification details and reopens the RFID database Scan Shield to refresh his memory of what he was querying for – information about the drone’s flight path before the bombing.
In the interface, Josh sees that 100 rows of data are corrupted. RedFlag’s AI-generated “Next Steps” feature indicates that Josh needs to clean his data before beginning his analysis. He is familiar with the suggested data cleaning process and clicks to begin. RedFlag’s AI recognizes the language of the unreadable data as hexadecimal code and suggests a way to translate the data. Josh accepts the translation and proceeds to the next step of the cleaning process, data deduplication. Josh sees the AI has flagged every duplicate in the dataset, not just those he believes are relevant to his analysis. He quickly edits the parameters to only select rows of data that are identical and RedFlag’s AI regenerates the selection. Josh accepts the changes and advances to the last step – removing missing data. RedFlag’s AI highlights three empty rows that can be removed to complete the data-cleaning process.
Finally, Josh scans the clean data to validate that the process was successful. Feeling confident, he resolves a RedFlag notification and reruns his initial flight path query on the newly cleaned data. RedFlag has made it easy to see inconsistencies and gaps to address in the data and simplified his data-cleaning work.
While he waits for this query to complete, Josh begins setting up a new query in a CCTV database commonly used in Oceania. He structures his query to search for CCTV footage from cameras within a five-mile radius of North Duke Street where the bombing occurred. As Josh writes the query, he receives a RedFlag notification alerting him of a spelling error. Josh expands the notification and sees that he has incorrectly spelled Macondo as “Makondo.” This has the potential to return results from outside Oceania and break compliance. Josh quickly corrects the spelling and runs the query.
As his results appear, Josh sees there’s a large chunk of video footage missing, potentially indicating that the cameras were not functional for multiple days. He clicks the gap area in the visualization and discovers that the software was unable to retrieve CCTV footage from April 6 through 11th. This means that any video footage of the drone’s flight path on the day of the bombing is missing. After ensuring that this gap is not due to an internal collection error, Josh checks the “Next Steps” in RedFlag which provides him with two possible solutions that may help him understand why the footage is missing. Josh sees that he can either expand his search to include commercial CCTV footage or start a new query for maintenance reports. After noticing that the maintenance report search will be faster, he proceeds with this option.
- Watch a video of the proposed RedFlag interface designed by Aimsley McDaniel, Sophia Milligan, and Leighann Vinesett: https://tae.ncsu-las.net/Josh
You Can’t Do That!
ComplAI for Michele, an experienced analyst who runs a non-compliant query
The third scenario describes what happens when an analyst queries something for which their intelligence agency does not have the authority to access; a non-compliant query. It is from the perspective of “Michele,” a seasoned, mid-career analyst. The students designed a widget that helps analysts like Michele maintain access compliance while searching for intelligence leads. Pronounced “comply,” ComplAI features alerts about data protection laws and the ability to automatically schedule appointments with legal experts.
Michele’s task is to find leads on Zendia’s military tactics, particularly the use of drones in armed conflict. Using the ComplAI interface, she searches a database of online transactions to look for links between SkyOne drone manufacturing and Zendia. Michele also types in a second request asking the AI to provide appropriate compliance recommendations. As she searches, she adds a justification note that she is analyzing purchase data for Zendian-manufactured drones to uncover security breaches and their link to a bombing using user interactions and time stamps.
While she’s analyzing the results of her search, ComplAI alerts her of an update to the European Union’s GDPR data protection law. The system flags Michele’s query as high risk for potential compliance violations. To learn more about this assessment, Michele uses the ComplAI chat. She inquires about regulatory guidelines related to purchase and transaction history. She asks a follow-up question asking for some examples of regulatory criteria in response. ComplAI suggests contacting a lawyer and displays an appointment booking module. She adds it to her calendar. It also points out the potentially non-compliant parts of her query and provides the source of the compliance breach. The AI suggests how to better frame her query and gives her an option to generate a risk-free compliant query, which she accepts and continues working.
- Watch a video of the proposed ComplAI interface designed by Soumya Batra, Aashka Patel, Leah Tatu, and Gabrielle Thorpe: https://tae.ncsu-las.net/Michele
Crossing the Streams
Spine for Miguel, an expert analyst who struggles to combine information from many different intelligence sources
Michele, Josh and Susie have all been working on different tasks related to intelligence gathering and analysis for the bombing in Macondo. Their team leader “Miguel” is an expert analyst, and LAS shared the following scenario with the design students describing how an expert analyst may approach synthesizing data from many places. The final group of design students created a prototype of a user interface for him called Spine.
At the start of the workday, Miguel receives an email from his agency’s headquarters that a bombing killed two people and injured others in the city of Macondo. He begins his investigation in the Spine software by setting the day’s goals and objectives. He selects the case type (terrorist attack) from the drop-down menu and inputs the case details. Miguel enters the time, date, location, and description of the bombing scenario for his team. These inputs inform the AI about the context of the case.
Miguel then adds case objectives and assigns them to junior analysts. He asks Josh to find suspicious activities through the networks in Macondo and Michele to analyze drone purchase data in Zendia. The AI recommends related datasets as useful starting points for the junior analysts. Miguel accepts some of these suggestions but he also adds a network dataset. He saves the goals, objectives and context and sends them to the junior analyst, Josh.
Josh receives a notification from Miguel asking him to look for suspicious network activity in the bombing area. Josh considers the recommended dataset and then he begins with the cellular network dataset. Josh inputs a prompt in natural language that generates a query using appropriate syntax. Josh requests data for WiFi connections in the cafe’s vicinity one day prior to the attack. He wants to identify potential suspects who utilize the network to connect to drone or other suspicious devices. Josh runs the query but he does not find any suspicious activity. Josh realizes that his query did not produce enough results because the time frame and the area were too narrow. So he broadens the parameters. He then discovers that a specific device has connected to multiple public WiFi networks within the specified time frame. This suspicious activity presents a significant lead. Excited, Josh makes sure to input the query, its justification, and findings, and then adds it to Spine.
While Miguel is running his own queries, some of his teammates queries appear in the team window labeled as outlier queries. The outlier flag could indicate a syntax error, a compliance break or perhaps a unique successful query. To overview the AI-identified outlier queries, Miguel cross-checks Josh and Michele’s queries.
Miguel examines one of Josh’s queries that is marked as an outlier. He discovers that Josh has formulated a unique approach to the query that yielded particularly promising results. He then reviews Michele’s query and prompts and discovers that her query produced broad results that might lead to compliance issues. He later mentors her in person regarding the compliance dangers.
Miguel finishes his daily task and starts crafting a team report. The AI generates documentation based on the input context, objectives, queries and findings. The results include executive summary, key observations and tradecraft analysis. Miguel can make edits to the AI-generated reports and cross verify them with the findings from his junior analysts to form a bigger picture of the investigation. Additionally the AI recommends refining future tradecraft and investigation processes providing Miguel with valuable results. Once Miguel is content with the report, he can seamlessly export it and share it with senior leadership at his intelligence agency.
- Watch a video of the proposed Spine interface designed by Parinita Das, Olha Novikova, and Vaishnavi Parni: https://tae.ncsu-las.net/Miguel
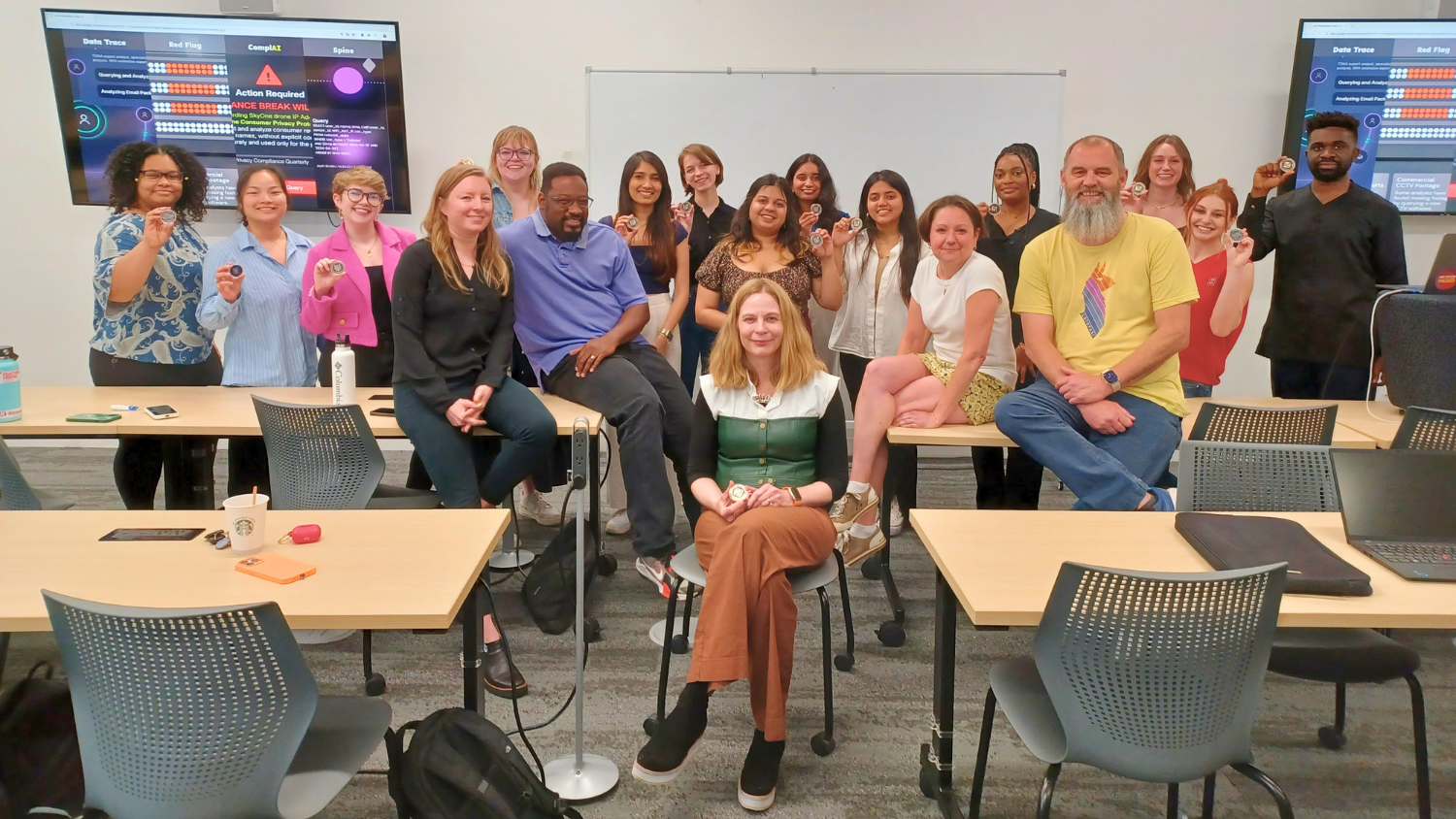
A Fresh Perspective
Ultimately, the students created four unique UX perspectives that can be used in future updates to real-world systems. Their final presentations show the true power of academia-government partnerships in safeguarding and protecting national security.
“This was a very enlightening experience working with the students and seeing fresh perspective in UX designs, as well as seeing their enthusiasm to work with government personnel to solve real-world problems,” a member of LAS said. “Often, UX is not really taken into account when designing tools that we use daily. The students took our feedback on board and this was evident during the evolution of their designs. The final products did not disappoint! I look forward to working with future students and who knows, the prototypes they envisage could turn into a real-world solution.”
About LAS
The Laboratory for Analytic Sciences is a partnership between the intelligence community and North Carolina State University that develops innovative technology and tradecraft to help solve mission-relevant problems. Founded in 2013 by the National Security Agency and NC State, each year LAS brings together collaborators from three sectors – industry, academia, and government – to conduct research that has a direct impact on national security.