Lost in Translation: Enhancing Operator Comment Workflows with New Technologies
LAS’s collaboration with UNC-Chapel Hill helps language analysts listen to audio recordings, translate them into English, and add operator comments to help the reader understand who or what the speaker may be discussing.
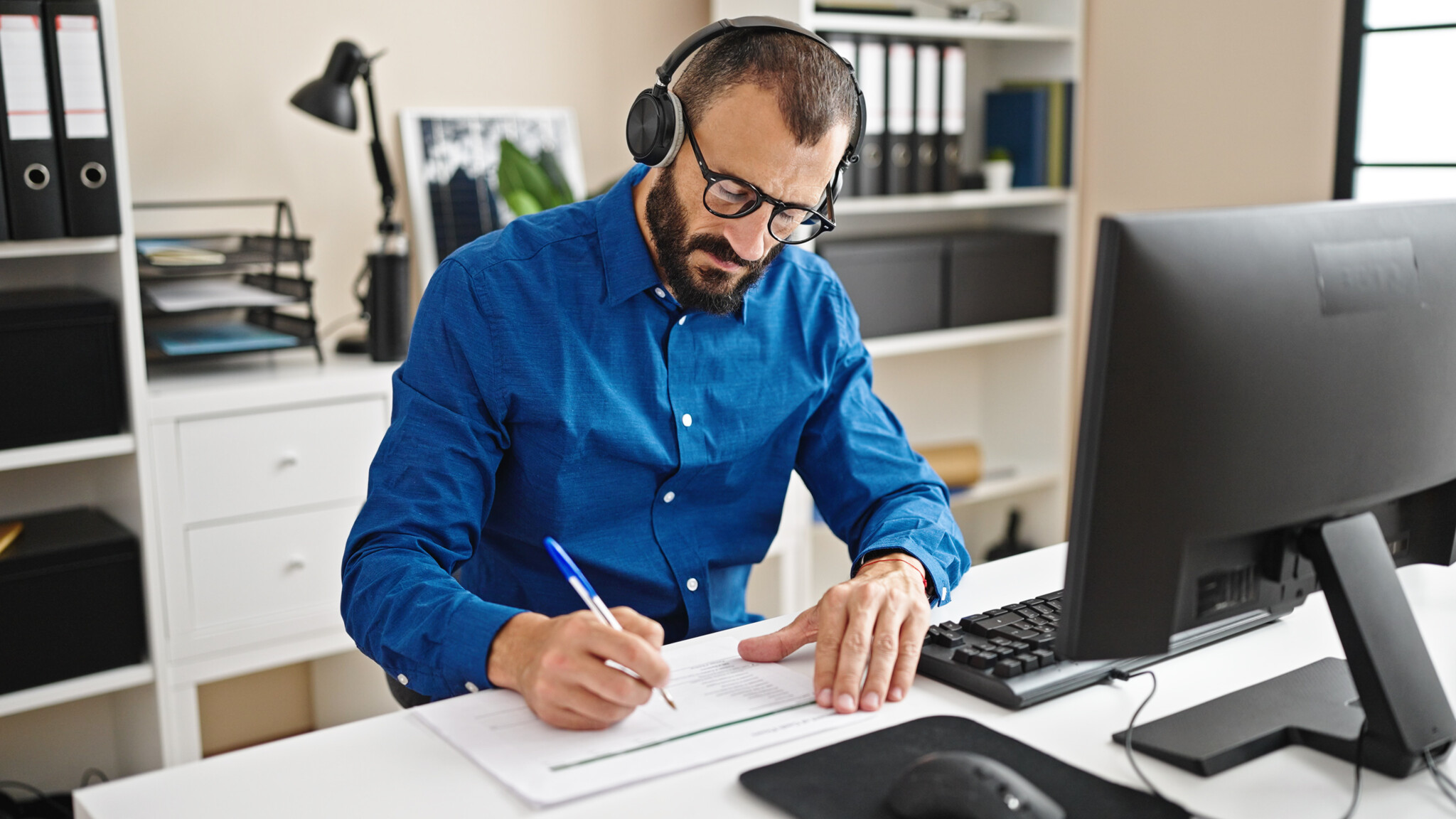
A name like “Dominique de Villepin” may be well known by French speakers, but generally unknown to English speakers; in this case, a translation of French text referring to him by name only may be rendered in English as “the former French Prime Minister Dominique de Villepin.” This process of making implicit information, which speakers of the source language can infer from context or situation, explicit for the consumers of the translation is known as “explicitation.” Ongoing collaboration between LAS and UNC-Chapel Hill’s School of Information and Library Science focuses on this tradecraft and shows that academia and the intelligence community can jointly accelerate the maturation and integration of technologies to enhance analytic workflows.
Explicitation: the process of making something explicit, or the fact of being explicit. It can also refer to the act of bringing something to light or verbalizing a concept or fact that has previously been hidden.
Tradecraft: The processes, tools and skills used for intelligence gathering.
Seeking Context Clues
In intelligence collection, there is often an abundance of implicit information that is understood by the foreign targets, but that would not be easily understood by others. This can be the result of speakers using local slang and idioms, or speaking with the assumption that the listener is aware of the context. It is the responsibility of multi-disciplined language analysts (MDLAs) to make this information explicit in their translations. MDLAs typically achieve this by adding “operator comments” or “OCs” to their translations. In the example above, a reference to “Dominique de Villepin” might be rendered as “Dominique de Villepin ((OC: the former French Prime Minister)).” Currently, making operator comments can be an ad-hoc and time-consuming process that requires MDLAs to decide when to make one, what information to include, and how to find that information. Once they make an operator comment in their transcript, the valuable information it contains will usually be seen only by the handful of other analysts who read the translation, then quickly get lost in a vast pool of unstructured data.
A Prototype to Help with Operator Comments
To improve this workflow, researchers at UNC School of Information and Library Science, led by Professors Rob Capra and Jaime Arguello, conducted a study in 2022 to understand the types of operator comments analysts make and the challenges they face when making them. Based on their findings, the UNC team developed a system to support analysts’ ability to make operator comments more efficiently and effectively. They conducted initial testing of the prototype with six intelligence community analysts in 2024, and updated the prototype based on that feedback.
Next Steps
For the remainder of 2024, UNC and LAS will also work on incorporating “predictive features” into the prototype to address the number one challenge cited by analysts who participated in the 2022 study: tracking down information to complete an operator comment. The features may use methods ranging from traditional information retrieval to generative AI to help auto-populate or suggest information to include in operator comments, or point analysts to where such information might be found.
In parallel with the development of the predictive features, the UNC team also planned a comparative study for later this year, in which they will evaluate and compare the quantity, quality, and variety of operator comment categories made by participants between conditions. They will also compare perceptions of workload, satisfaction, and difficulty. Meanwhile, LAS has recruited several teams of analysts to participate in a pilot using the UNC prototype in their operational workflows; the predictive features will also be integrated and tested in the second phase of the pilot. Feedback from analysts in the operational pilot, along with data from UNC’s comparative study, will guide intelligence community stakeholders in evaluating the value of systems that enable structured explicitation in translation workflows. This demonstrates how a combination of pilot testing in an operational environment and academic research can ensure timely and impactful outcomes.
About LAS
The Laboratory for Analytic Sciences is a partnership between the intelligence community and North Carolina State University that develops innovative technology and tradecraft to help solve mission-relevant problems. Founded in 2013 by the National Security Agency and NC State, each year LAS brings together collaborators from three sectors – industry, academia, and government – to conduct research that has a direct impact on national security.
- Categories: