Speech Emotion Recognition: Using High Arousal to Find Intriguing Conversations
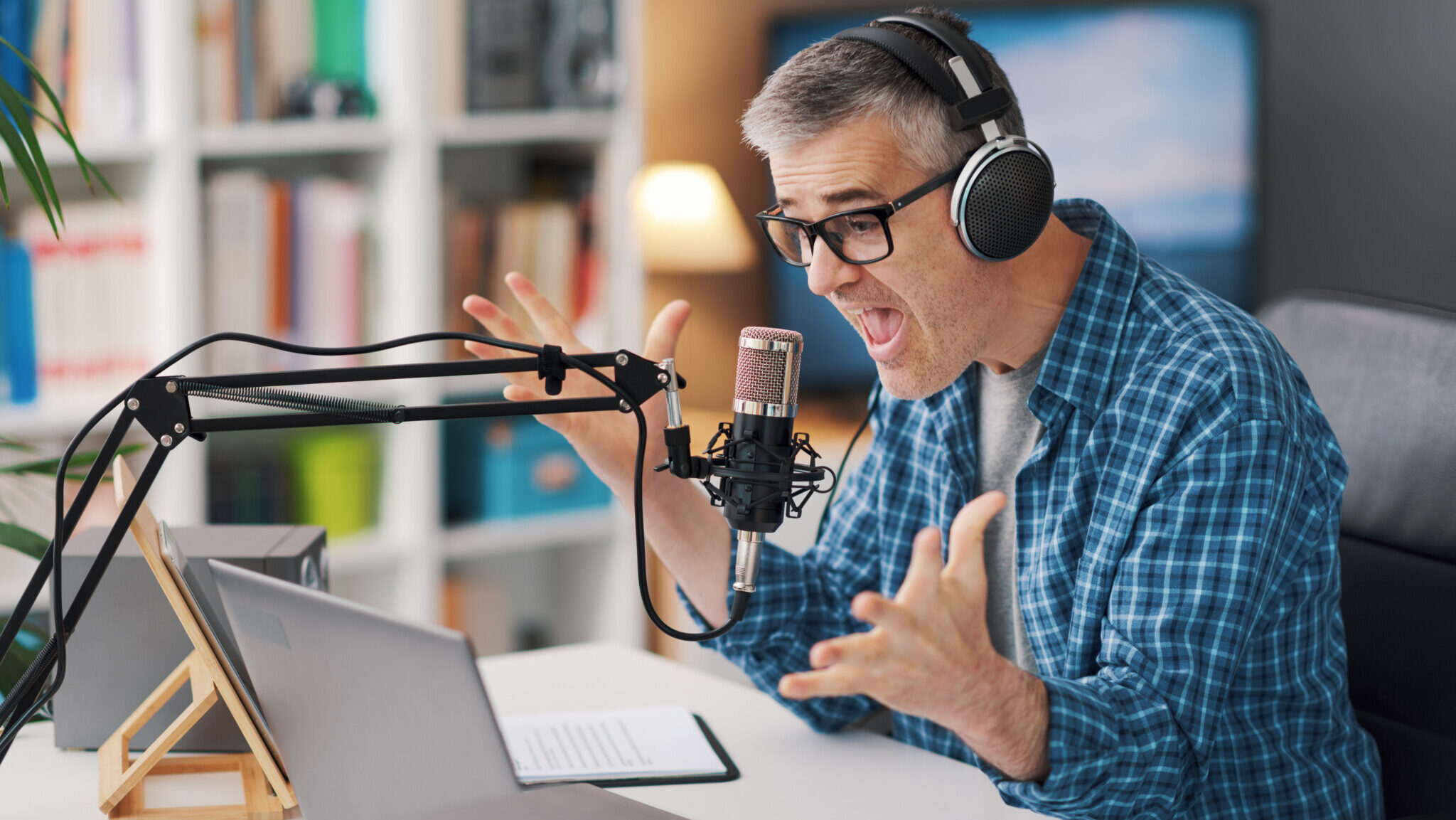
If we can search audio recordings by the emotions in a speaker’s voice, we could pinpoint a heated argument or surprise celebration.
A team of researchers at the Laboratory for Analytic Sciences is progressing on its multi-year goal to equip analysts with a tool that will provide labels indicating how people are talking. For the last three years, researchers and analysts have experimented with several machine-learning algorithms using a large naturalistic emotional speech dataset. The Multimodal Signal Processing podcast corpus was curated by LAS collaborator Dr. Carlos Busso and his team at the Erik Jonsson School of Engineering and Computer Science at the University of Texas at Dallas. This dataset contains tens of thousands of annotated segments from podcast recordings with Creative Commons licensing. It includes recordings of hundreds of speakers whose voices span the valence-arousal space — where valence describes whether an emotion is positive or negative. Arousal refers to the strength or intensity of an emotion.
The “Voice Characterization Analytics for Triage” project at LAS studies how we can use machine learning to determine the emotional dimensions present in voice recordings.
The following is an excerpt that researchers shared about the project’s goals and progress in 2023:
Speech Detectives
Last year, we created an analytic to detect high-arousal speech–when a speaker increases energy to express emotion, negative or positive. This year, we hope to test this algorithm on operational data.
The first step is to test our best-performing algorithm – a support vector machine (SVM) using wav2vec embeddings – on in-the-wild data to assess its accuracy outside a clean and friendly curated corpus.
What is in-the-wild data, you ask? It’s data that is not clean and friendly. This means it has not been manually reviewed to remove non-speech sounds or very short segments.
By assessing our algorithm on unfriendly data, we’ll not only be able to measure its accuracy on data that is different from our test data but also to harden both our code and pipeline to handle the wild operational environment we expect to encounter. Our current set of in-the-wild data consists of Presidents Nixon, Kennedy, and Johnson’s White House recordings and audio tracks from a video repository.
Unexpected Music
If you measure our progress by how many in-the-wild corpora we’ve assessed, we’re making very slow progress. We’re doing well, however, if you measure our progress by the number of unexpected problems we’ve solved. While we’re modifying our code to handle unexpected issues, we’re also redesigning our pipeline and trying to prepare for the differences in the operational computing environment we expect to encounter. We’re tackling issues such as how to handle singing, and speech with music in the background and we’re also determining how to install needed software on more restrictive operating systems. Additionally, we’re designing and testing Mongo database schemas that will allow for more efficient post-processing.
You may also be wondering how we’re assessing accuracy on our unlabeled in-the-wild corpora. That’s where the Infinitypool labeling tool comes in! After calculating postulated labels and scores on the automatically detected speech segments, we analyze the results and select a set for manual annotation. We determine our next steps based on our analysis of these manual labels. So far, this labeling is one of the primary ways to discover unexpected problems—like when we learned that the speech activity detection algorithm we’re using often false alarms on music.
Next Steps in Audio Labeling
During the years that we’ve been pursuing voice triage, we’ve discussed our ideas with many analysts in many offices. Because LAS provides us with such easy access to experienced and knowledgeable analysts, our primary method for assessing the validity of our ideas and algorithms is to interact directly with LAS analysts who are part of our team. The researchers on the team report quarterly to the analysts on the team to describe the experiments completed, what was learned from them, and what is planned next. When we have data to label, it’s both the analysts and the researchers who serve as annotators, thus providing a forum for discussing the algorithm’s performance and idiosyncrasies on a more practical level.
Although we haven’t made the progress we expected at the halfway point in 2023, we learned more than we expected, and we’re still hopeful we’ll meet our 2023 goal to evaluate algorithms on operational data.
—
About the Collaborators
Carlos Busso is a professor at the Erik Jonsson School of Engineering and Computer Science at the University of Texas at Dallas (UTD), where he leads the Multimodal Signal Processing (MSP) laboratory. His research interest is in human-centered multimodal machine intelligence and applications. His current research includes the broad areas of affective computing, multimodal human-machine interfaces, in-vehicle active safety systems, and machine learning methods for multimodal processing. His work has direct implications in many practical domains, including national security, health care, entertainment, transportation systems, and education.
About LAS
The Laboratory for Analytic Sciences is a partnership between the intelligence community and North Carolina State University that develops innovative technology and tradecraft to help solve mission-relevant problems. Founded in 2013 by the National Security Agency and NC State, each year LAS brings together collaborators from three sectors – industry, academia and government – to conduct research that has a direct impact on national security.
- Categories: