Enhancing the Art of Investigation: A GPT-based Creativity Support Tool for Analysts
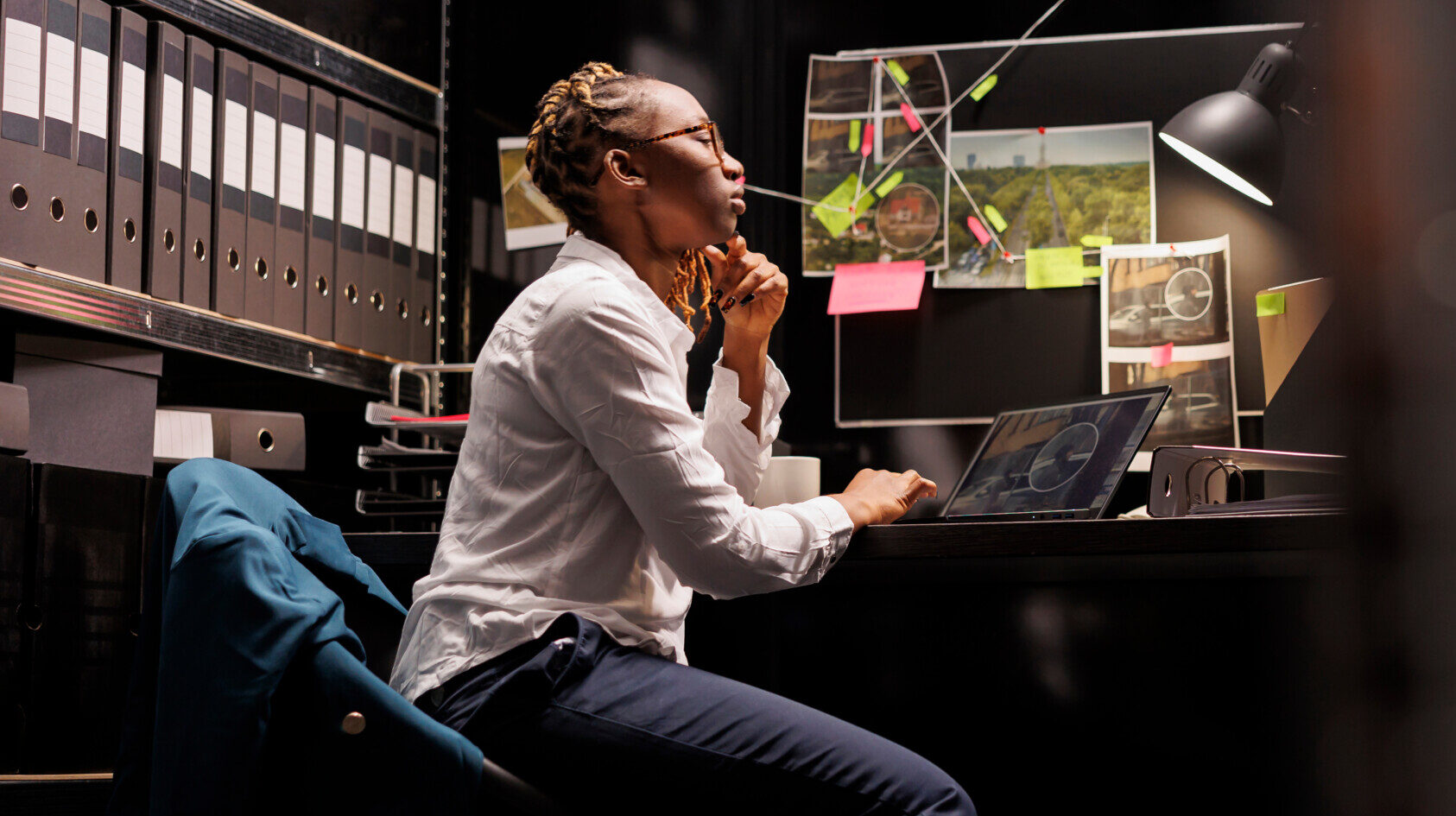
I paused in my work and glanced up at the clock– it was 3:00 a.m., and there seemed to be no end in sight. The desk was buried under a mountain of police documents and company records. Somewhere within this chaos, there had to be a clue. Proprietary software doesn’t just end up in a competitor’s hands by accident. I poured myself another cup of coffee, muttering, “There has to be a better way.”
Surely I had explored every possibility, carefully examining the connections between each piece of evidence… But what if my fatigued brain had overlooked something? What if some hypothesis remained unexplored? Suddenly, an idea struck me. I quickly switched tabs in my browser. I hadn’t used this new tool before, but I had heard about its promise from the developers on my team and it was worth a shot. I selected the name of my prime suspect from the dropdown and hit “query.” Leaning back in my chair, I waited for the model results to populate. If this new tech held the answer, well, I was up for promotion in six months and a big win like this would look great in my file.
The screen flashed, and a list of points loaded. I skimmed through the hypotheses quickly, finding that point #3 wasn’t correct. I hit the “thumbs down” icon; this would feed the negation of the incorrect statement back into the model and help it generate a better solution on the next run. I clicked “query” once again and waited as a new list of points loaded. I read through each one, scanning for errors, but this time they were all plausible. Highly plausible, in fact. These points confirmed my suspicions and provided evidence that I had previously missed. Satisfied, I started reading over the recommended documents and began typing up my report.
The scenario above is fictional, but the proposed solution is real and currently under development through LAS’s collaboration with Drs. Stephen Ware and Brent Harrison at the University of Kentucky. The project, titled “Inferring Domain Models to Help Analysts with Belief and Intention Recognition,” builds upon previous work to develop models of analyst workflows using the Insider Threat game created by the researchers. This year, the project employs large language transformers and models of causal reasoning to automatically infer a formal domain model from simple natural language event summaries. This process takes event summaries written in plain language and uses machine learning techniques to process the information contained within the summaries, with the goal of defining key concepts, rules, relationships, and causal patterns. Based on these inferences, an AI system can understand the context and provide intelligent suggestions to the analyst based on belief and intention recognition.
Within the platform itself, users are presented with evidence gathered by police after an insider attack at a small software company. The evidence is a large collection of documents, including emails, calendar entries, and server logs. The user’s task is to figure out which of the company’s employees stole its software. As users view each document, the underlying language model (GPT) automatically generates short summaries of the context and events. Users can add the relevant summaries into their personal repository of evidence, along with the option to write their own summaries for inclusion. As highlighted in the scenario above, users can browse the “Hypothesis” section of the tool and ask the AI to generate hypotheses about a suspect of interest. Any incorrect hypotheses can be corrected, which automatically feeds the negation of the statement back into the model for the next run.
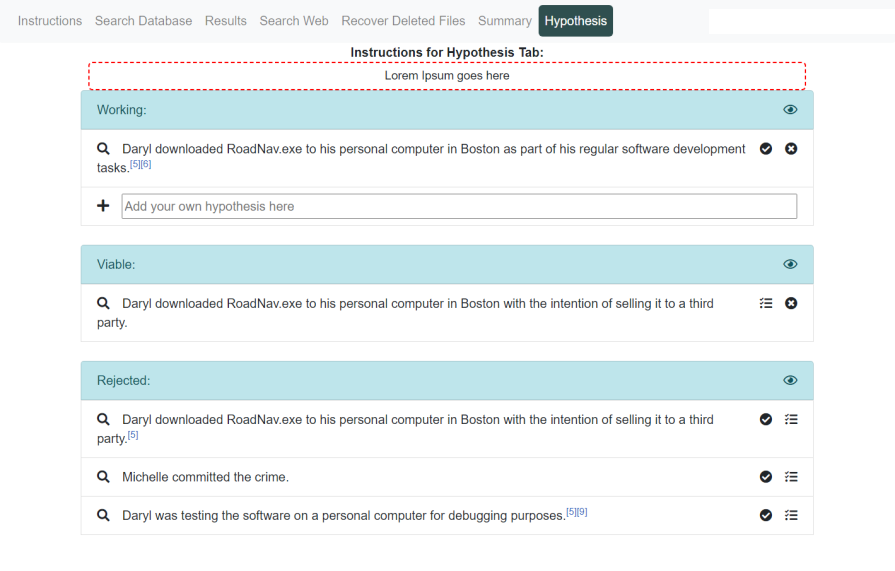
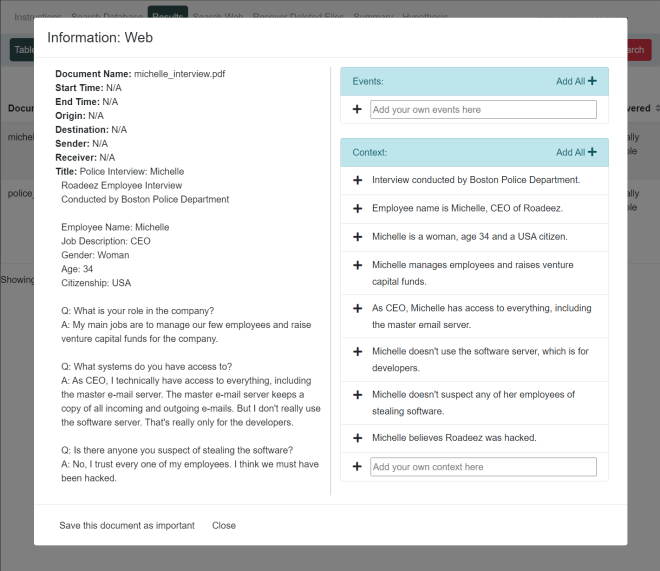
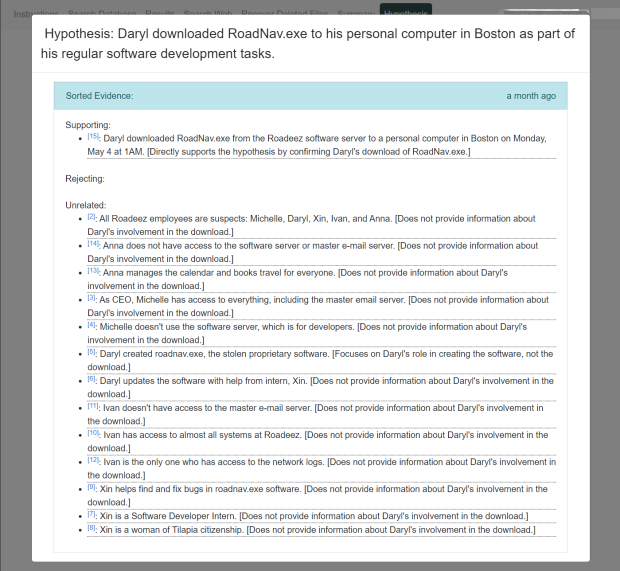
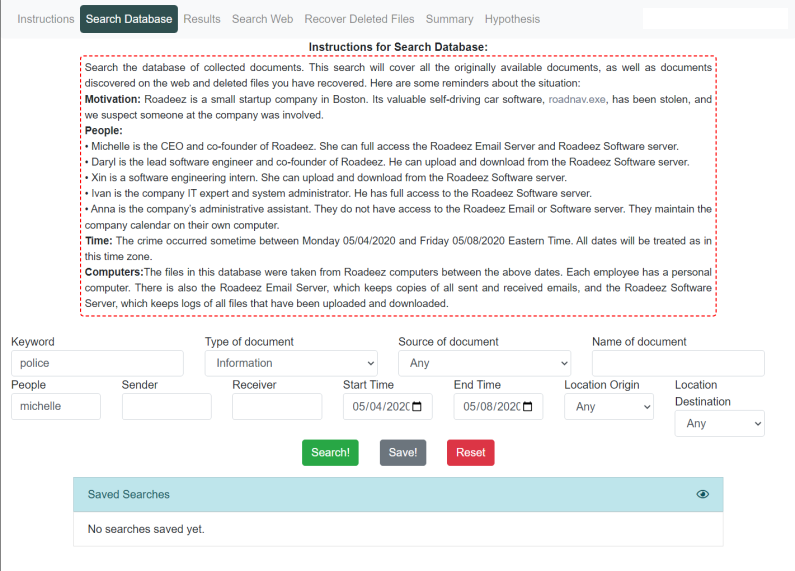
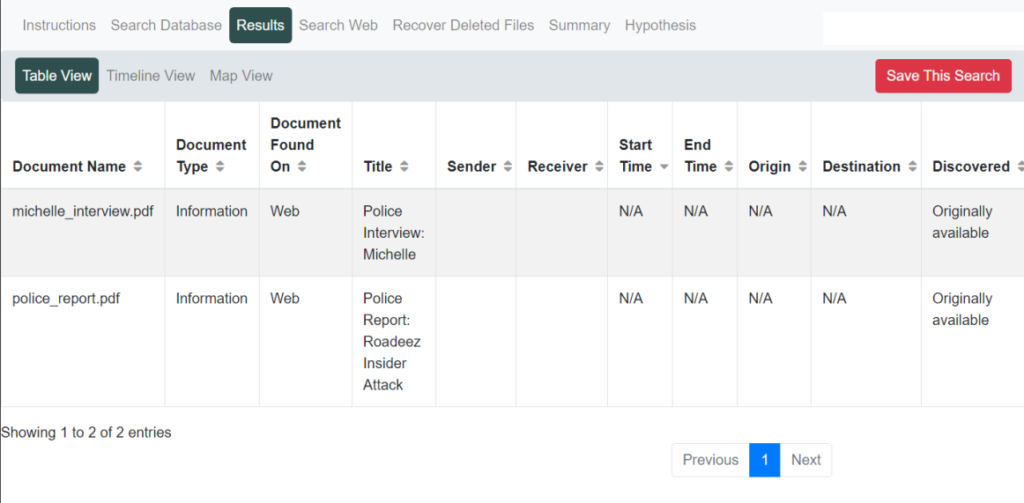
The project team’s work will continue with strengthening the construction of suggestions, making them more story-like in their formulation. Though GPT can be used to reason about future events and speculate about possible ways that events could have occurred, a story-like output is the necessary next step for enabling belief and intention recognition. While GPT is good at generating stories without constraints, it generally fails when asked to generate stories that have a particular ending or that include certain key events. Regular interactions with LAS analysts also provide the team with continuous feedback on the user interface and interpretability of the GPT outputs. We are enthusiastic about the progress of this project and see the potential for these models to be used as a powerful creativity support tool for analysts.
About the Collaborators
Brent Harrison is an assistant professor in the computer science department at the University of Kentucky College of Engineering. He specializes in game design and artificial intelligence, and intelligence with the ability to better understand the behavior and values of humans.
Stephen Ware is an associate professor in the computer science department at the University of Kentucky College of Engineering, where he teaches courses on artificial intelligence and game development. He is the director of the Narrative Intelligence Lab, which investigates how computers can use narrative to interact more naturally with people.
About LAS
The Laboratory for Analytic Sciences is a partnership between the intelligence community and North Carolina State University that develops innovative technology and tradecraft to help solve mission-relevant problems. Founded in 2013 by the National Security Agency and NC State, each year LAS brings together collaborators from three sectors – industry, academia, and government – to conduct research that has a direct impact on national security.
- Categories: