Survey of Metrics for Cognitive Load in Intelligence Community Settings
Christine Shahan Brugh (csbrugh@ncsu.edu), Eve Vazquez, James Peters, & Taylor Stone, North Carolina State University
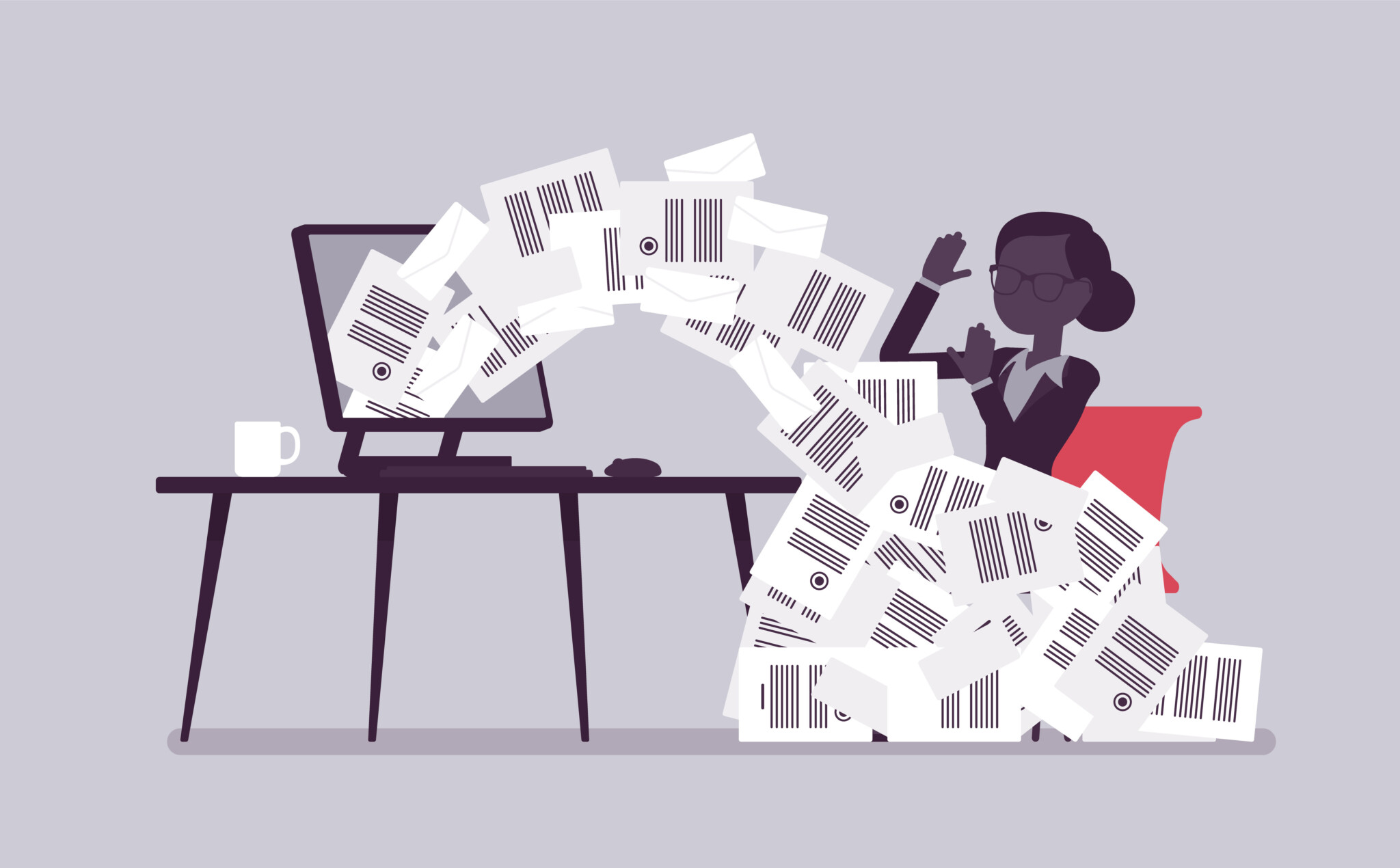
What is Cognitive Load and Why Does it Matter?
Cognitive load, understood as the demand placed by a task on the working memory of a person, is an important determinant of the performance of human-machine systems. Grounded in the framework of inherent limits to human’s memory capacity, high cognitive load has been shown to be associated with errors in task performance. For example, an analyst overloaded with information or task complexity might rely on less sophisticated ways of understanding the problem, such as cognitive biases or shortcuts.
In human-machine systems, if an analyst is overwhelmed by cognitive load, whether from information overload, multitasking, or complex interfaces, they might rely on shortcuts, biases, or less effective strategies to complete their work. This can lead to errors, delays, and reduced efficiency. As we seek to integrate sophisticated technologies into human-machine teams, measuring and managing cognitive load becomes crucial to ensuring that new tools are enhancing rather than hindering performance.
How Do We Measure Cognitive Load?
Understanding how cognitive load impacts performance is essential for designing more efficient systems. To understand how to measure cognitive load, we conducted a systematic review of extant cognitive load metrics. In doing so, we aimed to find reliable, valid metrics for cognitive load. We further sought to understand how different metrics might work in real-world, operational contexts, especially for intelligence analysts and other kinds of knowledge workers.
In total, we reviewed 125 articles published in academic journals between 1998 and 2024. From these articles, we derived a set of 129 unique metrics for cognitive load. These metrics fell into two broad categories: non-biometrics (e.g., questionnaires and task performance measures) and biometric (e.g., eye tracking, heart rate variability). We evaluated each metric on user burden, or how much effort a user would have to dedicate to completing the measure, and validation, or how well established the relationship is between the metric and cognitive load.
Key Findings
Non-biometric methods provide insights into cognitive load by analyzing user interactions with a device or system, behaviors (such as reaction time), and self-report data. These methods capture data through direct interaction with the user, instrumentation, and task performance outcomes. They range in level of user burden from None (for methods that rely on outputs the user is already producing, such as analyzing written text) to High (for measures in which the user must complete a questionnaire after the task is completed).
Three examples of types of non-biometric metrics are:
- Questionnaires – One of the most widely used metrics of cognitive load is the NASA-TLX, in which users answer seven questions about their perceived workload. Though these measures are subjective in nature, and rely on a user’s accurate self-assessment, most have substantial support through validation alongside other metrics of cognitive load. Metrics in this category range from Low to High user burden depending on the length of the questionnaire and how much time would be required of the user to complete it. A weakness of questionnaire type metrics is that they may lack nuance in measuring cognitive load over a very long, complex task, in which a user needs to interact with a variety of different tools.
- Task Performance – Many studies have sought to relate a user’s performance on an experimental task to cognitive load, often through employing multiple metrics simultaneously to be able to draw conclusions about the validity of measures such as speed or accuracy. These measures are highly task-dependent, and their level of validation ranges from Low to High. In contrast to questionnaires, these metrics typically do not involve any burden to the user, as they are collected passively as the user completes the task.
- Device Interactions and Movements – Interactions, such as keystrokes, mouse movements, or touch screen inputs, have been shown to be related to cognitive load. These metrics are often nuanced and task-specific, such as “contemplation-style pause behavior” which was defined as a set length of time without mouse movement in a task as compared to other patterns of movement in the task. Due to the specificity of these metrics, they are not often reproduced in more than one study, and thus are generally a low level of validation. Some exceptions for measures such as Reaction Time, which are studied more frequently. These metrics are also collected passively, as the user completes the task.
Biometrics provide objective measures of cognitive load by monitoring physiological responses. These metrics capture data from the human body, which reflects the automatic, physiological responses that take place due to cognitive load experienced by individuals in the context of an experimental task. They can offer advantages in terms of real-time data, and are more accurate due to their direct measurement. However, biometrics can be intrusive to users and may be difficult to implement in operational environments.
How to Use these Findings
All 129 metrics identified in our review were synthesized into a “catalog” of metrics for cognitive load. We include information mentioned above on user burden and validation, along with details on the task type, sample of participants in the study, and descriptions of each metric. Each metric includes a link to the published article for readers to engage further with the source material. However, with 129 metrics to choose from, selecting the right metric can still be a difficult task. Here are the main takeaways for integrating cognitive load metrics into a workflow:
- Identify the task: Start by breaking down the analytic work into components. What work is being performed at each stage? If a new tool is being developed, which part of the work, specifically, is it intended to enhance? Where are the bottlenecks or pain points for the users? These might indicate areas where cognitive load is particularly high and worth measuring.
- Find an analogous task: Using the task types described in the catalog, find one that most closely matches the analytic work. From there, look at the specific experimental tasks to find the best possible match. Applying metrics to a task for which they have not yet been tested might impact the validity of the measurement, so proceed with caution. However, if you are able to find a good match then you can be relatively confident that the metric will do a good job at assessing cognitive load.
- Select the metric(s) to implement: Once you’ve identified the analogous task, take a look at the metrics that have been used with that task. You might choose to implement one or multiple of them, depending on the associated user burden. If the metric can be collected without interrupting the user’s workflow, you might decide to also implement a questionnaire at the end of the task to get feedback directly from the user. Decide how much data you want to gather and what is feasible for follow-up analysis.
- Pilot test: Deploy the metric(s) with a small sample of users to ensure they are functioning as intended and not negatively affecting users.
- Deploy selected metrics: With your metrics selected and a data analysis plan in place, you can roll out the selected metrics to a wide sample of users and start getting feedback on cognitive load.
Conclusion
The continued evolution of human-machine teams and advancements in abilities of automated technologies makes the measurement and management of cognitive load an increasingly important priority. Selecting the right metrics for the task, user population, and reducing user burden as metrics are implemented is a step along the way to creating systems that improve efficiency and reduce mental workload for analysts.
This material is based upon work done, in whole or in part, in coordination with the Department of Defense (DoD). Any opinions, findings, conclusions, or recommendations expressed in this material are those of the author(s) and do not necessarily reflect the views of the DoD and/or any agency or entity of the United States Government.
- Categories: