“Enough is Enough”: Exploring Information Satiety in Knowledge Workers
Wenyuan Wang, UNC-Chapel Hill
David Gotz, UNC-Chapel Hill
Jordan Crouser, Smith College
Christine Brugh, NC State
Sue Mi K., LAS
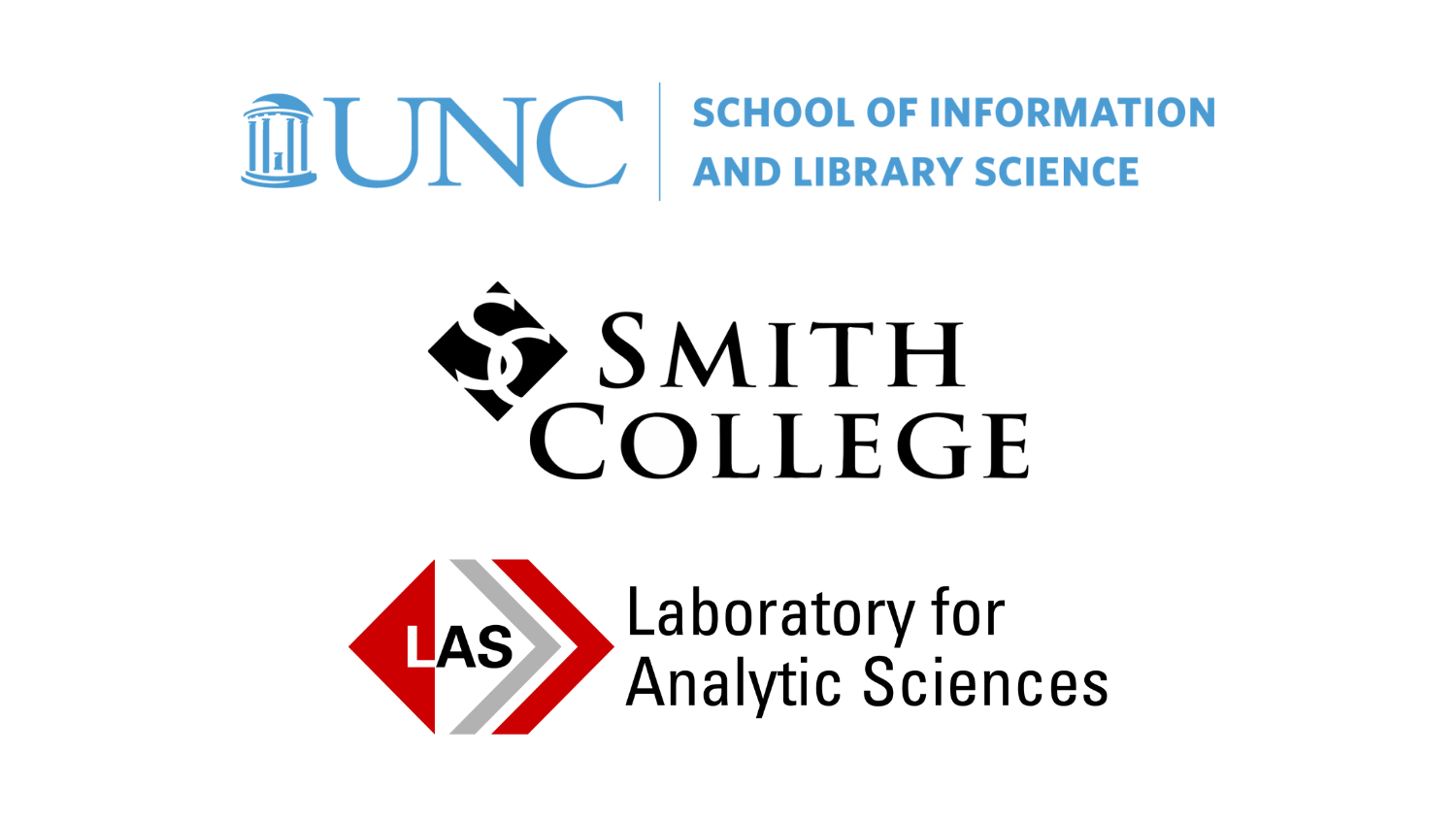
1.Overview
Today’s knowledge workers face a common dilemma: weighing the desire for more information against the natural constraints of time and other limited resources. Striking a balance between comprehensive analysis and timely decision-making is crucial in nearly every domain: the IC, industry, academia, journalism, and everyday life. To strike this balance, people must be discerning: how do we determine when we think we have “seen enough” to have confidence about a particular fact, pattern, or decision? Knowing when to dig deeper into the data, when to trust automation, and when to rely on our instincts is essential, yet we know relatively little about the underlying factors that influence information satiety.
This project takes a first step toward understanding the challenges and strategies people use to navigate the tension between the hunger for information and constraints on time and effort. We also explored how individual differences in personality and expertise may influence people’s information satiety.
More specifically, we conducted an online user study where people were asked to perform a simulated information task. The task required users to view a scatterplot and draw a best-fit line to represent the data. Users were financially incentivized to complete the task based on two competing metrics: the amount of data used (viewing more data earned a smaller payment) and accuracy (a more accurate best-fit line earned a higher payment). Participants, who also completed surveys to measure visualization literacy (Mini-VLAT) and individual personality profiles (BFI-10), performed multiple trials for tasks with two levels of difficulty.
Results from the study show evidence that participants’ performance in terms of accuracy and data use are influenced in distinct ways. While visualization literacy was a statistically significant factor in task accuracy, it was not a key factor influencing the amount of data used. The results suggest instead that information satiety (reflected in the amount of data used before drawing a best-fit line) is more strongly influenced by an individual’s personality profile. More specifically, people who look at more data tended to have higher agreeableness and openness.
The results may suggest that training (reflected by an increased visualization literacy) may yield improvements in task accuracy. At the same time, that training may not alter information satiety which may depend more on individual personality traits. These preliminary findings offer some hints about the factors that may influence peoples’ choices about when they’ve “seen enough,” but more study is needed to understand the mechanisms and broader set of factors that may influence information satiety. The remainder of this post provides more details about our research.
2. Experimenting with a Simple Problem First
Information satiety is a complicated phenomenon and is potentially influenced by many factors. This makes studying satiety in the “real world” a difficult endeavor. To get started with our research, we chose to focus on a relatively simple information task with well-defined properties. By learning about satiety in this simple environment, we can hope to study in the future how our findings may generalize to more complex contexts.
More specifically, our experiments focus on a simple task in which users are asked to draw a best-fit line for data in a basic two-dimensional scatter plot. Users are given control over how many data points they see before drawing their guess at a best-fit line, and they are incentivized to both
- use less data and
- be as accurate as possible.
This type of simple information problem is easy to explain to participants without special training. Moreover, it can be mathematically modeled to identify what statistically constitutes “enough” information to perform the task to a given accuracy. This theoretically grounded point of enough information lets us study how and when peoples’ point of information satiety varies from a statistically grounded point of sufficiency.
We recognize, of course, that these types of simple problems are highly constrained and not representative of many of the information tasks that people do every day. However, we argue that findings from studying information behavior within this simple context can potentially shed light on how people behave in increasingly realistic and complex information problems.
3. Study Design
We designed an interactive web-based online user study that was compatible with the CloudResearch platform which provides recruitment and payment services for study participants. This section describes the basic experimental task that users were asked to perform, the data used as stimuli in the experiments, and a detailed overview of the study protocol.
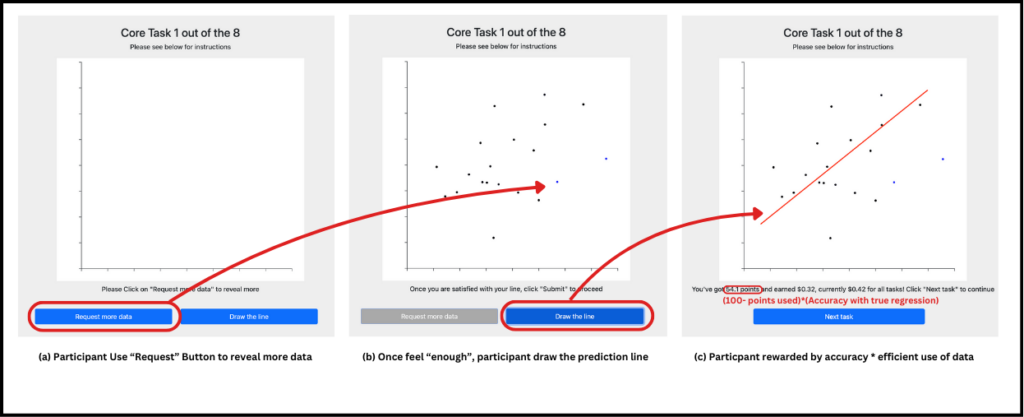
Experimental Task:
Participants were asked to perform eight experimental tasks, each following a similar design but showing different data. For each task, a participant views a scatterplot of data points and is asked to draw the best-fit line representing a linear trend seen within the scatterplot. The task begins with the participant viewing an empty scatterplot devoid of data points. The participant is then required to repeatedly request additional data by clicking on a button below the chart. Each click of the button adds additional data points to the plot. Once the participant decides that they have seen enough data to draw a best-fit line, they click a second button to lock in the number of data points. They can then draw a line directly over the scatterplot to represent their best estimate of the task solution. The participant clicks a final button to indicate that they are done drawing their estimate of the best-fit solution. This process is illustrated in Figure 1.
Critically, during a training phase that occurs before the experimental tasks, participants are introduced to the basic task as well as the task’s incentive structure. More specifically, participants are informed that they will earn a monetary bonus that is higher if they perform the task accurately. At the same time, they are informed that the bonus will be reduced every time they request more data. These contradictory incentives force participants to balance a trade-off between requesting more data to allow a more accurate answer, while minimizing data usage to receive a higher bonus payment.
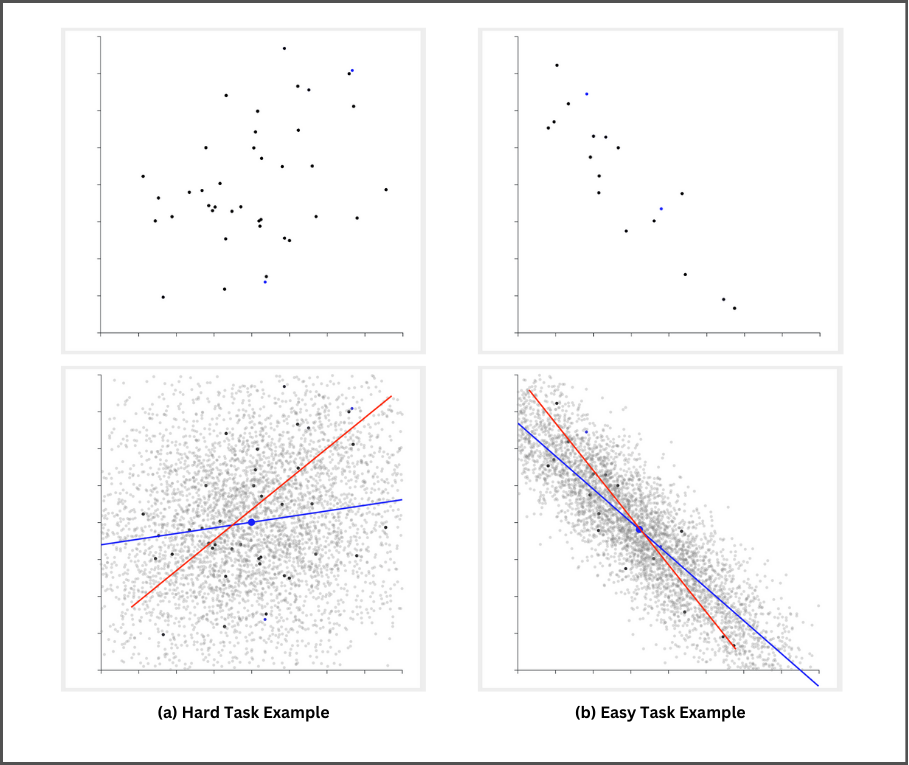
Dataset: An artificially generated dataset is used throughout the study. This allows for the careful control of the amount of correlation present in the scatterplot data as well as the slope and intercept the best-fit trendline. Perhaps most critically, the degree of correlation present in the generated dataset serves as a proxy for task difficulty in our experiments. More specifically, we created “easy” and “hard” datasets comprising 5000 data points each and with correlation values of 0.3 and 0.8, respectively. This is illustrated in Figure 2. The large dataset size (5000 data points) was far in excess of the number of data points required to perform the task, resulting in what was essentially an “infinite” dataset for participants to view before deciding it was time to draw the best-fit line. Consequently, the data viewed by participants for any given tasks was only a very small portion of the entire dataset. Each of the two generated datasets was transformed to use in 4 distinct tasks (4 easy tasks and 4 hard tasks) through the application of a series of rotations and translations. To mitigate potential learning effects, the order of tasks and the presentation of transformed datasets were pseudo-randomized when assigned to participants.
Dependent Variables: In total, each participant performed 8 experimental tasks for which we measured two dependent variables: (1) the accuracy of the participant’s best-fit line with respect to the overall distribution of data (not just the data displayed in the chart, but against all 5000 points), and (2) the number of data points viewed before drawing the line.
Independent Variables: The study involved six independent variables. Five of these were personality traits as measured by the BGI-10 survey which contains 10 questions for participants to self-report their sub-categorical factors. The five traits include Extraversion, Agreeableness, Conscientiousness, Neuroticism, and Openness. The questions capture measurements for each trait using a 5-point Likert scale.
The sixth and final independent variable was visualization literacy. This was measured through the Mini-VLAT instrument which asks users to answer time-limited questions about 12 different types of visualizations. This provides a score on the scale of 0-12 which has been shown in the literature to reflect a participant’s degree of visualization literacy.
Protocol: Participants recruited from CloudResearch were provided with an overview of the project and asked to provide their consent for participating. They were then given a Mini-VLAT test before introduced to the study tasks and financial incentives via a tutorial. After the tutorial, participants completed three practice tasks which were similar to the actual tasks except that participants were shown a true regression line as well as the full underlying dataset at the end of the practice task. This was done to help ensure that participants understood how more data was available and could help them answer the task more accurately if they wished to use it.
Participants then completed the 8 main tasks (4 easy and 4 hard) in a pseudo-random order. User interaction data, along with data use and accuracy, were recorded for each task. Then participants were asked to complete two brief surveys: demographic questionnaire and the BFI-10 personality assessment. Finally, participants were presented with a questionnaire containing five open-ended questions to gather their reasoning regarding information engagement within and beyond the scope of the study.
Participants were compensated with a based pay of $2.50 plus a flexible amount that ranged from $0 to $4.80 depending on their performance with the main tasks. The study took about 20 minutes to complete.
4. Study Results
In total, 80 participants were recruited for the study. A review of the task completion data revealed that 12 participants exhibited signs of hasty completion or lack of engagement. Consequently, these participants were excluded from the analysis. As a result, we had a final sample size of 68 participants. Each participant completed 4 easy tasks and 4 hard tasks, resulting in a total of 272 observations for each task type in terms of data usage and accuracy.
To analyze the results, we performed multi-linear regressions to characterize the influence of the six independent variables over both data usage and accuracy. We performed separate regressions for easy and hard tasks. Results for participants’ data usage are presented in Tables 1 and 2 for easy and hard tasks, respectively. Statistically significant results are marked in green. For both easy and hard tasks, participants’ data usage is correlated to their agreeableness (Coef = 4.519, p<0.05, and Coef = 6.325, p<0.05, respectively).
Coef | Std Error | t value | p-value | |
Intercept | 9.983 | 15.635 | 0.639 | 0.524 |
Extraversion | -0.483 | 1.925 | -0.251 | 0.802 |
Agreeableness | 4.519 | 1.894 | 2.386 | 0.018 |
Conscienctiousness | -1.993 | 2.171 | -0.918 | 0.359 |
Neuroticism | 1.817 | 1.939 | 0.937 | 0.349 |
Openness | 3.014 | 2.180 | 1.383 | 0.168 |
Mini-VLAT | -0.847 | 0.798 | -1.062 | 0.289 |
Coef | Std Error | t value | p-value | |
Intercept | -17.487 | 25.132 | -0.691 | 0.487 |
Extraversion | -0.950 | 3.095 | -0.307 | 0.759 |
Agreeableness | 6.325 | 3.045 | 2.077 | 0.039 |
Conscienctiousness | -0.488 | 3.489 | -0.140 | 0.889 |
Neuroticism | 4.549 | 3.116 | 1.460 | 0.146 |
Openness | 7.733 | 3.504 | 1.636 | 0.103 |
Mini-VLAT | 0.058 | 1.283 | 0.045 | 0.964 |
Results from the regressions for participants’ task accuracy are presented in Tables 3 and 4 for easy and hard tasks, respectively. For easy tasks, accuracy was correlated with Openness (Coef = 0.019, p<0.05) and Visual Literacy scale (Coef= 0.008, p<0.05), but on hard tasks the relationship with openness was no longer statistically significant.
Coef | Std Error | t value | p-value | |
Intercept | 0.689 | 0.067 | 10.280 | 0.000 |
Extraversion | -0.012 | 0.008 | -1.478 | 0.141 |
Agreeableness | 0.001 | 0.008 | 0.069 | 0.945 |
Conscientiousness | 0.009 | 0.009 | 1.007 | 0.315 |
Neuroticism | -0.000 | 0.008 | -0.036 | 0.972 |
Openness | 0.019 | 0.009 | 2.033 | 0.043 |
Mini-VLAT | 0.008 | 0.003 | 2.423 | 0.016 |
Coef | Std Error | t value | p-value | |
Intercept | 0.352 | 0.108 | 3.252 | 0.001 |
Extraversion | 0.006 | 0.013 | 0.418 | 0.676 |
Agreeableness | 0.026 | 0.013 | 1.943 | 0.053 |
Conscientiousness | -0.014 | 0.015 | -0.944 | 0.346 |
Neuroticism | 0.018 | 0.013 | 1.373 | 0.171 |
Openness | 0.027 | 0.015 | 1.758 | 0.080 |
Mini-VLAT | 0.146 | 0.006 | 2.641 | 0.009 |
5. Discussion
In this study, we investigated the effects of personality traits and visual literacy on participants’ data usage (as a proxy for information satiety) and accuracy during a simple best-fit line task.
The results regarding data usage suggest that information satiety is most strongly impacted by a participant’s agreeableness. Interestingly, agreeableness as a trait can often be associated with difficulty making tough decisions. This is aligned with the observation that more data is viewed by people who score highly in this particular trait.
Equally important, visual literacy is not associated with differences in information satiety. If we view literacy as a proxy for training (i.e., more training with visualizations will result in higher visualization literacy), this result suggests that training with the task alone is perhaps unlikely to be an effective mechanism for changing a user’s information satiety behavior. In contrast, visual literacy does have a statistically significant relationship with task accuracy. This seems to suggest that people can be trained to be better at a task and perform it more accurately. However, the same expertise that leads to more accurate performance doesn’t mean that more experienced people are comfortable working with less data.
These observations raise an important question: how we can influence a user’s sense of satiety if not by training? This is a critical question to address in future work, and we hypothesize that design choices in the user interface can potentially influence users’ sense of satiety. We hope to conduct future experiments to test and quantify this hypothesis.
As previously described, our study design focused on a simple task in which there was a statistically grounded definition of “enough” data to complete the task within a given level of accuracy. Table 5 shows the sample size statistically required to perform the task of drawing a best-fit line within a specific significance level (e.g., alpha = 0.05) and desired power (e.g., beta = 0.8).
Power / Correlation | Hard Task: Corr = 0.3 | Easy Task: Corr = 0.8 |
Beta = 0.95 | 289 | 41 |
Beta = 0.8 | 174 | 25 |
Participants’ Average Data Use | 35.4 | 26.4 |
As seen in Table 5, participants generally reach the statistically sufficient threshold to accurately draw the best-fit line for easy tasks. They request on average 26.4 data points when statistically they need between 25-41 depending on the desired level of accuracy. However, in hard tasks users fall far short. Participants are more likely to adopt satisficing strategies, stopping early to avoid the effort of looking at the required number of data points. They may hastily conclude that no accurate prediction can be made and give up, or they may overfit to insufficient data. Qualitative feedback gathered during our experiment suggests a mixture of both of these forces are at play. For example, some suggested that they “had a general direction of which way the dots were going” or that “adding data doesn’t to provide a lot perspective”, while others suggested they “just use my intuition and hope for the best.” This suggests that for hard tasks, there is more opportunity and perhaps need for intentional efforts to design interfaces that guide people to use more data before reaching a conclusion.
6. Limitations and future work
In the analysis presented above, each task performed by a participant was treated as an independent observation. However, our study design had each participant perform 8 tasks (4 easy and 4 hard). This aspect of our study design means that the observations are not strictly independent. A multi-level modeling approach (or other similar hierarchical analysis methods) can account for the fact that each participant performed multiple tasks. However, with our current sample size (68 participants), such an analysis is underpowered and does not detect statistically significant effects. We are currently working towards a larger study with a larger sample size to allow us to perform this type of analysis.
Additionally, our study gathered a rich set of qualitative data from our end-of-study questionnaires. We are in the process of analyzing that data via thematic analysis. This effort should provide additional insights that may help explain some of the quantitative findings in our study.
This material is based upon work done, in whole or in part, in coordination with the Department of Defense (DoD). Any opinions, findings, conclusions, or recommendations expressed in this material are those of the author(s) and do not necessarily reflect the views of the DoD and/or any agency or entity of the United States Government.
- Categories: